AI in demand forecasting: Use cases, benefits, solution and implementation
Businesses face constant challenges in anticipating customer demand due to volatile and unpredictable market conditions. Companies are often left grappling in the dark, trying to understand the next big trend that can affect market conditions. In such a scenario, demand forecasting emerges as a strategic tool, helping businesses stay competitive. At its core, demand forecasting involves providing insights into future customer demand patterns based on past sales data and present market conditions.
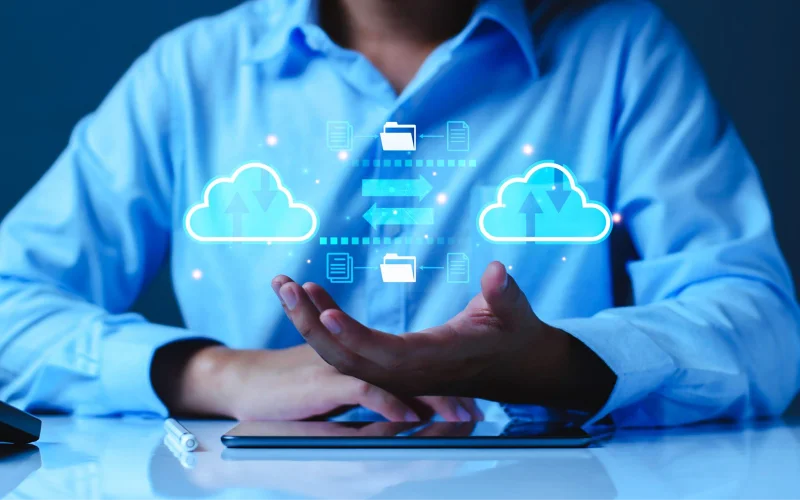
Traditionally, demand forecasting methods were carried out manually and primarily relied on historical and current order data and statistical analysis. While effective in stable market conditions, these approaches often struggle to accurately predict demand in volatile environments. Artificial Intelligence (AI) and Machine Learning (ML) address these limitations by processing and analyzing large volumes of data quickly. They take into account various factors such as seasonality, promotions, and market dynamics, delivering forecasts that are more accurate and adaptable to changing conditions. By handling complex data that would overwhelm manual methods, AI and ML enhance forecasting accuracy and help businesses anticipate shifts in consumer behavior more effectively. It’s evident in the findings of Gartner’s survey. A staggering 45% of companies have already incorporated machine learning into their demand forecasting processes, and the trend only seems to grow. The reasons are clear: enhanced accuracy, optimized supply chain dynamics, better financial planning, and reduced risks.
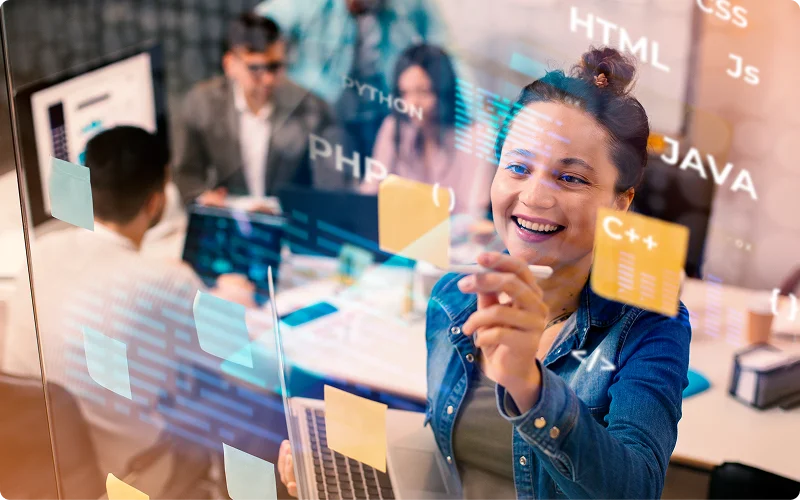
What is AI-enabled demand forecasting?
Traditional forecasting methods, known as “demand-driven” approaches, have long relied on order data to predict future inventory needs. However, these methods often fall short in today’s unpredictable market. The challenges? The problems include overstocking when demand drops and understocking during unexpected surges. These issues can strain resources and become a financial burden for businesses.
Enter AI-enabled demand forecasting, a game-changing approach that combines technology with strategy to improve logistical operations. But what is AI in demand forecasting, and how does it transform businesses?
AI in demand forecasting leverages artificial intelligence (AI) to refine and optimize the forecasting process. Instead of only relying on past sales data, it considers a broader range of information—current market trends, social media activity, online reviews, and more. This integrated approach does not just collect data; it analyzes it, identifies patterns, anticipates changes, and provides predictive insights that traditional models cannot match.
Moreover, the statistics speak for themselves. According to McKinsey Digital, AI-enhanced forecasting reduces errors in supply chain networks by 30 to 50%. This increased accuracy leads to a significant drop—up to 65%—in lost sales due to out-of-stock situations. Transportation and warehousing costs are projected to decrease by 5-10%, while supply chain administration expenses are expected to be reduced by 25-40%. The broader economic impact is substantial, adding an estimated $1.2 trillion to $2 trillion in value to manufacturing and supply chain planning.
Beyond AI, integrating machine learning into supply chain management enhances these benefits. Traditional models like ARIMA (Auto Regressive Integrated Moving Average) and exponential smoothing, which rely solely on historical data, are outperformed by the real-time insights machine learning provides. These algorithms actively learn from data, adapting with each new data set. They consider factors like demographics, social media trends, resulting in forecasts that are more accurate and nuanced.
A notable advantage of machine learning in demand forecasting is its ability to tackle challenges posed by new products. Traditional models stumble here due to a lack of historical data. However, machine learning overcomes this by finding similar products from the past, analyzing their lifecycle, and using that information to predict the new product’s path.
AI in demand forecasting isn’t just a technological upgrade—it’s a paradigm shift. By combining AI and machine learning with strategic foresight, businesses can navigate the complexities of the modern supply chain with confidence and precision. This approach is not just the future of inventory management; it sets the gold standard.
How does AI for demand forecasting work?
Incorporating AI into demand forecasting transforms traditional approaches by leveraging advanced Large Language Models (LLMs) and connecting them with an organization’s expansive data sets. This innovative approach enhances data analysis and generates deep insights, facilitating precise forecasting and helping businesses optimize inventory, manage supply chains, and align production with market trends.
This architecture leverages various components to streamline the AI-driven demand forecasting process. Here’s a step-by-step breakdown of how it works:
Data sources
Effective demand forecasting relies on diverse and comprehensive data inputs, including:
- Historical sales data: Detailed records of past sales performance, which help identify trends and patterns essential for forecasting.
- Market trends data: Insights into current market conditions and emerging trends that affect consumer demand.
- Consumer behavior data: Data from customer interactions and purchasing behaviors to understand preferences and predict future buying trends.
- Competitor activity: Insights into competitor promotions, pricing strategies, and product launches, which can influence market dynamics.
Data pipelines
Data from the above sources are processed using sophisticated data pipelines that handle their ingestion, cleaning, and structuring, preparing the data for detailed analysis.
Embedding model
An embedding model processes this prepared data, transforming it into a format that AI systems can effectively analyze. Popular models from providers like OpenAI, Google, and Cohere are utilized for this purpose.
Vector database
The generated vectors from the embedding model are stored in a vector database such as Pinecone, Weaviate, or PGvector, enabling efficient querying and rapid retrieval.
APIs and plugins
APIs and plugins like Serp, Zapier, and Wolfram play a key role by connecting different components and enabling additional functionalities, such as accessing extra data, integrating with necessary tools or platforms or performing specific tasks with ease.
Orchestration layer
The orchestrating layer is critical in managing the workflow. ZBrain is an example of this layer that simplifies prompt chaining, manages interactions with external APIs by determining when API calls are required, retrieves contextual data from vector databases, and maintains memory across multiple LLM calls. Ultimately, this layer generates a prompt or series of prompts that are submitted to a language model for processing. The role of this layer is to orchestrate the flow of data and tasks, ensuring seamless coordination across all demand forecasting operations.
Query execution
The data retrieval and generation process begins when the user submits a query to the demand forecasting app. These queries relate to future market demands, product performance, or inventory needs.
LLM processing
Once received, the app transmits the query to the orchestration layer. This layer retrieves relevant data from the vector database and LLM cache and sends it to the appropriate LLM for processing. The choice of LLM depends on the nature of the query.
Output
The LLM generates an output based on the query and the data it receives. This output can come in various forms, such as expected product demand, market reactions to upcoming changes, and recommendations for supply chain adjustments.
Demand forecasting app
This specialized app delivers AI-generated forecasts in an accessible format, enabling business planners and supply chain managers to make informed decisions quickly.
Feedback loop
User feedback on the LLM’s output is another important aspect of this architecture. The system incorporates user feedback to continuously improve the accuracy and relevance of the LLM’s outputs.
Agent
AI agents step into this process to address complex problems, interact with the external environment, and enhance learning through post-deployment experiences. They achieve this by employing advanced reasoning/planning, strategic tool utilization, and leveraging memory, recursion, and self-reflection.
LLM cache
Tools like Redis, SQLite, or GPTCache are used to cache frequently accessed information, improving the response time of the AI system.
Logging/LLMOps
Throughout this process, LLM operations (LLMOps) tools like Weights & Biases, MLflow, Helicone and Prompt Layer help log actions and monitor performance. This ensures the LLMs operate at peak efficiency and evolve consistently through ongoing feedback mechanisms.
Validation
A validation layer is employed to validate the LLM’s output. This is achieved through tools like Guardrails, Guidance, Rebuff, and LMQL to ensure the accuracy and reliability of the information provided.
LLM APIs and hosting
LLM APIs and hosting platforms are essential for executing demand forecasting tasks and hosting the application. Depending on the requirements, developers can select from LLM APIs offered by companies such as OpenAI and Anthropic or opt for open-source models. Similarly, they can choose hosting platforms from cloud providers like AWS, GCP, Azure, and Coreweave or opt for opinionated clouds like Databricks, Mosaic, and Anyscale. The choice of LLM APIs and cloud hosting platforms depends on the project’s needs and preferences.
This structured flow offers a comprehensive overview of how AI improves demand forecasting processes. It utilizes AI tools and advanced data analysis to streamline operations, enhance accuracy, and support proactive decision-making in response to market demands.
Use cases of AI demand forecasting across industry verticals
Historical data analysis
AI can analyze historical sales data, taking into account factors such as seasonality, holidays, and promotions, to identify patterns and trends. This analysis helps retailers understand past consumer behavior and make more informed predictions for future demand.
Predictive analytics for inventory management
AI algorithms can analyze real-time data from various sources, including point-of-sale systems, online transactions, and social media. By incorporating this data, retailers can predict future demand more accurately, enabling them to optimize inventory levels and avoid stockouts or overstock situations. Inventory demand forecasting using machine learning enhances the inventory management process by identifying patterns and trends that traditional methods might miss, leading to even greater precision in inventory planning.
Market trends and external factors
AI-powered demand forecasting tools can continuously monitor and analyze external factors such as social trends. By incorporating these insights into the forecasting models, retailers can adjust their predictions based on factors that may impact consumer behavior.
Customer segmentation
AI can analyze customer data to segment the market based on preferences, buying behavior, and demographics. By understanding the distinct needs of different customer segments, retailers can tailor their inventory and marketing strategies to meet specific demand patterns for each group.
Dynamic pricing optimization
AI-powered pricing algorithms can adjust prices dynamically based on real-time demand fluctuations, competitor pricing, and other market variables. This helps retailers maximize revenue and respond quickly to changes in consumer behavior.
Promotion planning
AI can assist in planning and optimizing promotional activities by predicting the impact of promotions on demand. Retailers can identify the most effective promotional strategies and allocate resources accordingly to drive sales without overcommitting inventory.
Supply chain optimization
AI can enhance demand forecasting by integrating with supply chain systems. By considering factors such as lead times, transportation constraints, and supplier reliability, retailers can ensure a more synchronized supply chain that aligns with predicted demand patterns.
Demand forecasting for transportation planning
A logistics company needs to optimize its transportation network based on anticipated demand for different routes and modes of transport. Demand forecasting ML models analyze historical shipment data, customer orders, and market trends. AI helps in predicting future transportation needs, allowing the company to optimize routes, allocate resources efficiently, and ensure timely deliveries.
Warehouse space optimization
A warehouse manager aims to optimize space utilization based on expected changes in product demand. AI-driven demand forecasting models analyze SKU-level data, order history, and market trends. This information assists in predicting which products will experience increased demand, enabling the warehouse to adjust storage configurations, allocate space accordingly, and improve overall inventory management.
Supplier collaboration and production planning
An electronics manufacturer relies on multiple suppliers for components and needs to align production with varying demands for finished goods. AI-driven supply forecasting tools analyze historical order data, production lead times, and supplier performance metrics to forecast component demand accurately. This enables the manufacturer to collaborate with suppliers, streamline production planning, and avoid shortages or excess inventory at different stages of the supply chain.
Demand forecasting for inventory replenishment
A retail chain with multiple locations needs to replenish inventory at each store based on local demand patterns. AI algorithms analyze sales data, historical inventory levels, and external factors (such as local events or promotions) to forecast demand at individual stores. This allows for optimized inventory replenishment strategies, minimizing stockouts and reducing excess inventory carrying costs.
Demand forecasting for cross-border logistics
An international logistics provider wants to optimize customs clearance processes and shipping routes based on anticipated changes in demand for cross-border shipments. AI-driven supply forecasting tools analyze global trade data, regulatory changes, and historical shipping patterns to forecast demand for specific international routes. This enables the logistics provider to optimize customs clearance procedures, allocate resources efficiently, and improve overall cross-border logistics efficiency.
Customer behavior analysis
Through customer behavior analysis, AI leverages data from diverse channels, including online platforms and customer service interactions. Predictive models meticulously discern patterns and trends in customer preferences, enabling manufacturers to gain invaluable insights. With this knowledge, manufacturers can strategically adapt production volumes and refine product offerings, ensuring a proactive response to anticipated changes in customer demand and fostering a more agile and customer-centric manufacturing approach.
Seasonal demand fluctuations
By leveraging AI models that thoroughly analyze historical sales data across various seasons, predictive analytics emerges as a potent tool for manufacturers to forecast seasonal demand fluctuations precisely. Using these insights, manufacturers can strategically optimize their production schedules, fine-tune inventory levels, and efficiently manage staffing resources. This proactive approach enables businesses to adeptly meet peak demand during specific seasons, ensuring operational efficiency and customer satisfaction.
Dynamic pricing strategies
AI-powered demand forecasting tools play a pivotal role in the manufacturing sector by seamlessly integrating market conditions, competitor pricing, and historical sales data. Through predictive models, it intelligently recommends optimal pricing strategies aligned with precise demand forecasts. This dynamic pricing approach empowers manufacturers to adapt swiftly, ensuring they can maximize revenue by aligning prices with current market dynamics and efficiently meeting customer demand.
Customized product demand forecasting
AI, leveraging data on customized product orders and customer preferences, employs predictive models to accurately forecast demand for specific configurations and customizations in the manufacturing sector. By harnessing this insight, manufacturers gain the ability to optimize production processes, ensuring they can efficiently meet the dynamic demand for personalized products. This tailored approach not only enhances customer satisfaction but also streamlines production resources, contributing to a more agile and responsive manufacturing ecosystem.
Investment portfolio management
AI-powered forecasting solutions play a pivotal role by meticulously examining historical market data, and sentiments expressed in news sources. Utilizing this wealth of information, predictive models crafted by AI technologies forecast the potential performance of diverse securities and investment instruments. Armed with these insights, investment managers are empowered to proactively optimize and rebalance portfolios, strategically navigating anticipated market shifts. This integration of AI-driven forecasting enhances precision in decision-making, enabling investment professionals to stay ahead in the dynamic landscape of financial markets.
Customer product preferences
By leveraging artificial intelligence, banks meticulously scrutinize customer transaction histories, behavior patterns, and interactions with a spectrum of banking products. Through predictive modeling, these institutions can anticipate the demand for specific financial products, ranging from loans and credit cards to savings accounts. Armed with these insights, banks adeptly tailor their marketing strategies and product offerings, aligning them with individual customer preferences. This proactive approach not only enhances customer satisfaction but also ensures that the financial institution remains agile and responsive to evolving demands in the dynamic landscape of banking services.
ATM and branch optimization
By harnessing the power of AI, banks leverage historical transaction data and analyze foot traffic patterns at ATMs and branches to enhance operational efficiency. Through predictive models, these institutions accurately forecast peak usage times and strategic locations for their ATMs and branches. Armed with this foresight, banks can proactively optimize resource allocation, ensuring not only sufficient staffing but also the availability of cash resources during high-demand periods. This intelligent approach to resource management allows banks to enhance customer service, minimize wait times, and streamline operations based on real-time demand insights.
Personalized banking services
By using AI in financial forecasting, banks can analyze individual customer data, such as spending habits, financial goals, and major life events. With advanced predictive models, banks can understand and predict personalized service preferences. This allows them to offer tailored services, including customized financial planning, investment advice, and personalized loan options, all aligned with each customer’s unique needs. This AI-driven personalization enhances customer satisfaction and helps banks meet the evolving demands of a diverse clientele.
Predictive maintenance
AI algorithms leverage historical vehicle maintenance data to predict component failures and replacement patterns. By accurately anticipating when specific parts are likely to fail, automakers can proactively plan and schedule the production of replacement parts. This predictive maintenance approach enhances operational efficiency, reduces downtime, and ensures the timely availability of replacement components, contributing to improved customer satisfaction and cost-effective maintenance strategies.
Supply chain optimization
AI plays a pivotal role in demand forecasting for the automotive industry by analyzing factors such as raw material availability, transportation delays, and production bottlenecks. By understanding these variables, AI systems can predict potential disruptions and assist automakers in optimizing their supply chain to meet forecasted demand efficiently. This integration of demand forecasting with supply chain optimization ensures a more accurate alignment of production capabilities with anticipated market needs, ultimately improving overall operational efficiency.
Market trends and consumer behavior analysis
AI-powered forecasting solutions are instrumental in analyzing social media, online reviews, and various customer feedback sources to discern emerging market trends and evolving consumer preferences. By extracting insights from this data, automakers gain a nuanced understanding of current and future market demands. This knowledge empowers them to dynamically adjust production plans, ensuring that products align closely with the preferences and expectations of consumers, thereby enhancing their competitiveness in the automotive market.
Customization and personalization
AI’s capacity to analyze data on individual customer preferences and buying behavior is pivotal in predicting demand for specific vehicle configurations and features. This predictive capability empowers automakers to customize their production processes, aligning them with the demand for personalized and tailored vehicles. As a result, automakers can offer a more personalized and diverse range of products, meeting the specific desires of their customers and potentially gaining a competitive edge in the market.
Dealer and inventory management
AI plays a crucial role in optimizing dealer inventories by analyzing sales data, regional demand patterns, and seasonal variations. By providing actionable insights into which vehicle models and configurations are in high demand in specific regions, AI empowers automakers and dealers to manage their inventory more effectively. This proactive approach ensures that dealers stock the right products in the right quantities, minimizing excess inventory and aligning supply with localized demand dynamics.
Medication and vaccine demand forecasting
By scrutinizing historical prescription data, disease prevalence, and vaccination trends, AI employs predictive models to anticipate the demand for specific medications and vaccines. This foresight equips healthcare providers to optimize their inventory, production processes, and distribution channels effectively, ensuring timely and sufficient availability of essential pharmaceuticals to meet anticipated demand. This application of AI streamlines healthcare operations, enhancing responsiveness to the dynamic healthcare landscape.
Hospital bed and staffing optimization
By analyzing historical patient admissions, seasonal trends, and patterns of disease outbreaks, AI forecasts the demand for hospital beds and staffing needs. This foresight enables hospitals to proactively reallocate resources, ensuring optimal facilities and healthcare personnel availability during periods of heightened demand. This AI-driven approach enhances the efficiency of healthcare resource management, ultimately improving patient care and response capabilities during peak demand scenarios.
Medical equipment and supplies forecasting
By scrutinizing usage patterns, maintenance records, and external influences on medical equipment, AI conducts predictive modeling to anticipate demand for diverse medical devices and supplies. This enables healthcare facilities to streamline procurement and storage processes, mitigating the risk of shortages or excess inventory. The result is a more cost-effective and efficient management of resources in healthcare settings.
Personalized medicine demand forecasting
By analyzing genetic data, patient records, and treatment outcomes, AI facilitates personalized medicine demand forecasting. Through predictive models, the technology anticipates the demand for individualized therapies and targeted treatments. This empowers healthcare providers to tailor treatment plans according to expected patient needs and advancements in personalized medicine, ensuring a more precise and patient-centric approach to medical care.
Booking management
AI-powered demand forecasting is instrumental in optimizing booking management within the travel and hospitality industry. By accurately predicting demand for flights, hotels, and rental cars, businesses can implement dynamic pricing strategies. This involves adjusting prices in real-time based on fluctuations in demand, maximizing revenue while maintaining optimal occupancy rates. Furthermore, precise demand forecasts enable effective inventory management, preventing overbooking or underbooking of resources.
Seasonal planning
Anticipating seasonal demand variations is crucial for successful operations in the travel and hospitality sector. AI-driven forecasting can help businesses identify peak travel periods, enabling them to allocate resources and adjust staffing levels accordingly strategically. By analyzing historical data and external factors, businesses can optimize resource utilization and reduce operational costs. Additionally, understanding demand patterns within specific market segments allows for targeted marketing efforts and tailored service offerings.
Customer experience
Leveraging AI for demand forecasting enhances customer experience in several ways. By predicting customer preferences and behavior, businesses can deliver personalized recommendations, increasing customer satisfaction and loyalty. Furthermore, AI enables the identification of customer segments with similar demand patterns, facilitating targeted marketing campaigns and customized service offerings. Additionally, forecasting wait times for services like check-in and baggage claim helps manage customer expectations and improve overall service delivery.
Streamlining demand forecasting workflow with generative AI
Generative AI transforms demand forecasting by automating and enhancing data analysis. By leveraging advanced algorithms, it streamlines data collection, model training, and forecast adjustments, leading to more accurate and actionable insights. Here is the detail about how generative AI streamlines demand forecasting workflow:
Steps Involved | Sub-Steps Involved | Generative AI application |
---|---|---|
Collect data |
|
|
Insights generation |
|
|
Steps Involved | Sub-Steps Involved | Generative AI application |
---|---|---|
Demand review |
|
|
Sales forecasting |
|
|
Plan adjustment |
|
|
Steps Involved | Sub-Steps Involved | Generative AI application |
---|---|---|
Inventory optimization |
|
|
Replenishment strategy |
|
|
Inventory cost management |
|
|
Steps Involved | Sub-Steps Involved | Generative AI application |
---|---|---|
Resource planning |
|
|
Check resource usage |
|
|
|
|
How to implement AI in demand forecasting?
Implementing AI in demand forecasting involves several key steps to ensure successful integration and accurate predictions. Here is a general guide on how to implement AI in demand forecasting:
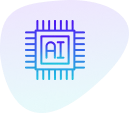
Define objectives and scope
Clearly define the objectives of implementing AI in demand forecasting. Identify specific areas, products, or services where AI will be applied. Set measurable goals and key performance indicators (KPIs) to evaluate success.
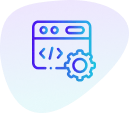
Data collection and preparation
Gather historical data on relevant variables, including sales, customer behavior, market trends, and external factors. Clean and preprocess the data to ensure accuracy and consistency. Address missing values, outliers, and any other data quality issues.
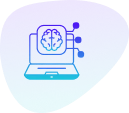
Choose the right AI algorithms
Select appropriate AI algorithms based on the nature of the demand forecasting problem. Common algorithms include linear regression, decision trees, neural networks, and time series models (e.g., ARIMA or LSTM).
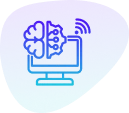
Feature engineering
Identify and create relevant features that can enhance the performance of the AI models. This may involve transforming or combining existing variables to provide more meaningful inputs for forecasting.
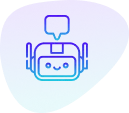
Model training
Split the historical data into training and validation sets. Train the AI model using the training set and validate its performance on the validation set. Adjust hyperparameters, such as learning rates or hidden layer sizes, to optimize the model’s accuracy.
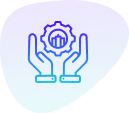
Integration with IT infrastructure
Ensure that the AI model can be integrated into existing IT infrastructure seamlessly. This may involve collaboration between data scientists and IT professionals to deploy the model on the organization’s servers or cloud platforms.
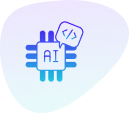
Real-time data integration
Implement mechanisms to feed real-time data into the AI model. This could involve setting up data pipelines or utilizing APIs to ensure that the model receives the latest information relevant to demand forecasting.
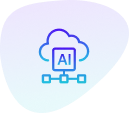
Validation and testing
Validate the AI model’s accuracy and performance using historical data that was not used during the training phase. Test the model under different scenarios to assess its robustness and reliability.
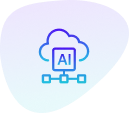
Iterative improvement
Continuously monitor the performance of the AI model and gather feedback from users. Use this feedback to make iterative improvements to the model over time.
Comprehensive enterprise services & support
Factors influencing the accuracy of AI-based demand forecasting
Factors influencing the accuracy of AI-based demand forecasting encompass various aspects crucial for precise predictions. These include:
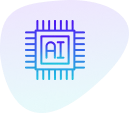
Data quality
The accuracy of AI-based demand forecasting hinges on the data quality used for training models. Inaccurate or outdated data can lead to flawed predictions, impacting inventory management and supply chain operations.
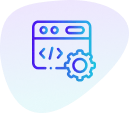
Historical data reliance
While historical data guides AI models of existing patterns, solely relying on it can limit adaptability to unexpected changes. Supplementing historical data with relevant real-time information enhances forecasting accuracy.
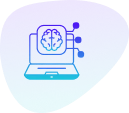
Model complexity
The complexity of AI models affects forecasting accuracy. Models must strike a balance—too simplistic may overlook crucial patterns, while excessive complexity risks overfitting and poor performance on new data.
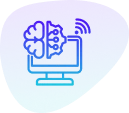
Real-time data integration
AI platforms leverage real-time data processing capabilities to analyze complex variables swiftly. This enables immediate adjustments to forecasts based on evolving conditions or recent developments in the supply chain.
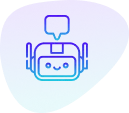
Continuous learning
Machine learning models refine their predictions over time through continuous learning from operational data. This iterative process enhances forecasting accuracy by incorporating new insights.
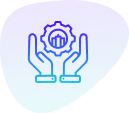
Minimization of human error
Automation in AI-based forecasting reduces human error, enhancing overall efficiency and precision. By replacing manual processes with automated routines governed by AI, biases and inconsistencies are mitigated, leading to more accurate forecasts.
Key considerations for building AI-based demand forecasting systems
In addition to the core steps of building an AI-based demand forecasting system, it’s crucial to consider various factors to ensure its effectiveness, transparency, and long-term viability. Here are some considerations to incorporate into the development process:
Focus on product-market fit
- Understanding user needs: Prioritize solving a well-defined problem that aligns with the needs of the target user group.
- Start narrow, expand gradually: Before scaling, establish product-market fit by beginning with a specific department, product category, or geographic region.
- Iterative development: Continuously gather user feedback to refine the system and ensure it meets their evolving needs.
Transparent communication
- Explain AI capabilities: Clearly communicate how AI-based forecasting works and its benefits to stakeholders, including management, employees, and customers.
- Address concerns: Through open and transparent dialogue, acknowledge and address any concerns or misconceptions about AI, such as job displacement or privacy issues.
- Feedback loop: Establish a feedback loop to encourage communication and collaboration between data scientists, domain experts, and end-users.
Data-driven decision making
- Utilize data insights: Base decisions on empirical evidence and insights derived from data analysis to enhance forecasting accuracy and operational efficiency.
- Identify and overcome biases: Use data to identify and mitigate biases in traditional forecasting methods, ensuring more objective and reliable predictions.
- Ongoing monitoring: Regularly monitor key performance indicators and data quality to inform decision-making and identify opportunities for improvement.
Scalability and future-proofing
- Design for scalability: Build a flexible and scalable infrastructure that can accommodate increased data volumes, model complexity, and user demand over time.
- Plan for growth: Anticipate future needs and potential expansion by designing the system with scalability regarding technology and business processes.
- Adaptability: Ensure the system can easily integrate new data sources, adapt to changing market conditions, and incorporate advanced forecasting techniques as they emerge.
Explainability
- Interpretability of models: Ensure that AI-based forecasting models are transparent and understandable, allowing stakeholders to interpret how predictions are made and trust the results.
- Provide insights: Explain forecast outcomes, highlighting the factors and variables that contribute to each prediction to enhance understanding and facilitate decision-making.
- Build trust: Transparency and explainability build trust among users, increasing confidence in the system’s reliability and fostering adoption.
Data security
- Protect sensitive data: Implement robust security measures to safeguard sensitive information, including customer data, proprietary algorithms, and business insights.
- Compliance with regulations: Adhere to data protection regulations and industry standards to ensure legal and ethical handling of data, mitigating risks of data breaches or privacy violations.
- Regular audits: Conduct regular security audits and assessments to identify vulnerabilities and address any potential threats or weaknesses in the system.
How does DeepSurge’s generative AI platform optimize demand forecasting processes?
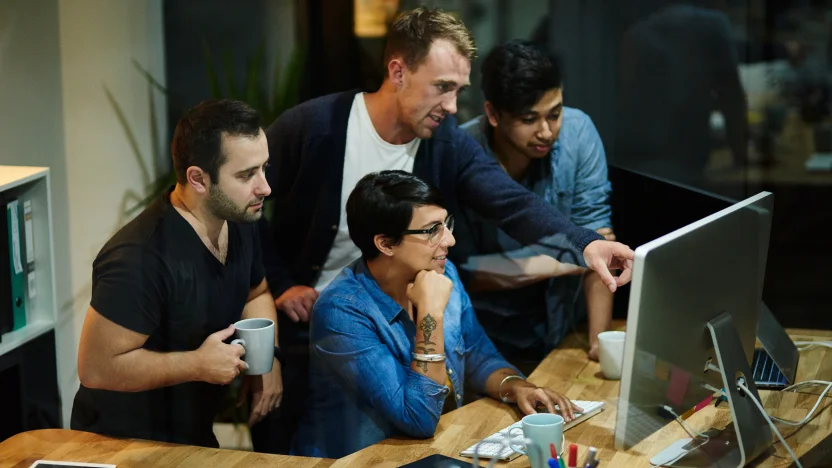
AI-driven demand forecasting in retail
Effective retail success and customer satisfaction hinge on precise inventory optimization and demand forecasting. Achieving the delicate balance between maintaining optimal inventory levels and accurately predicting product demand is intricate. ZBrain simplifies these processes through automation, gathering pertinent data like historical sales, current inventory, product catalog, seasonality, and supplier lead times. An automated exploratory data analysis (EDA) phase extracts valuable insights and transforms textual data into numerical representations using advanced embedding techniques. ZBrain’s seamless transformation provides businesses with precise insights, enhancing decision-making. Upon receiving an inventory optimization and demand forecasting query, ZBrain fetches relevant data based on specifications. This data and the query are then analyzed by ZBrain’s AI-driven algorithms and the chosen Language Model (LLM). This dynamic analysis ensures accurate demand forecasting, optimized inventory levels, and efficient supply chain management.
AI-driven demand forecasting in logistics
ZBrain addresses the complexities of inventory management and warehouse planning by utilizing advanced AI algorithms for accurate demand forecasting. By automatically collecting sales history, inventory levels, market trends, and external factors, ZBrain creates a comprehensive dataset. Through an Exploratory Data Analysis (EDA), the platform uncovers insights, identifies variations, and transforms textual data into numerical representations using advanced embedding techniques. This enhances the accuracy of demand forecasting by capturing subtle nuances. When users request a demand forecasting report, ZBrain fetches relevant data based on query requirements and processes it using the chosen Language Model (LLM) for dynamic report generation. The resulting report, rich in insights on projected demand scenarios, trends, and influencing factors, is meticulously parsed. Essential information such as demand projections, trends, and inventory requirements is extracted, ensuring a concise, accurate, and professionally presented output for informed decision-making.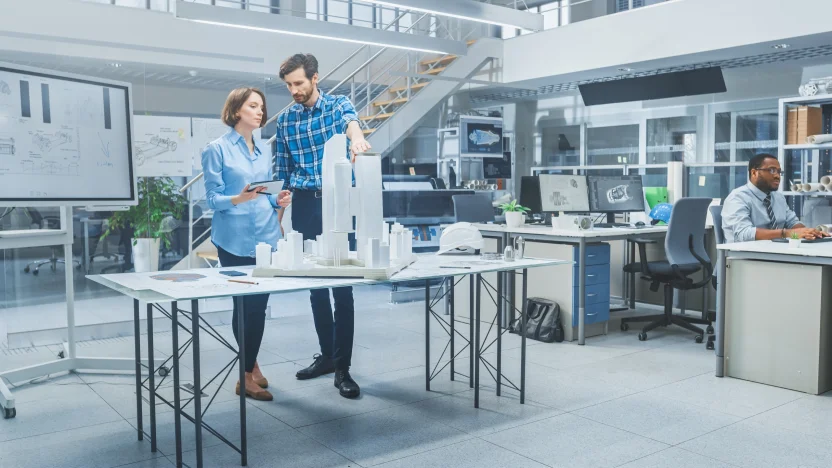
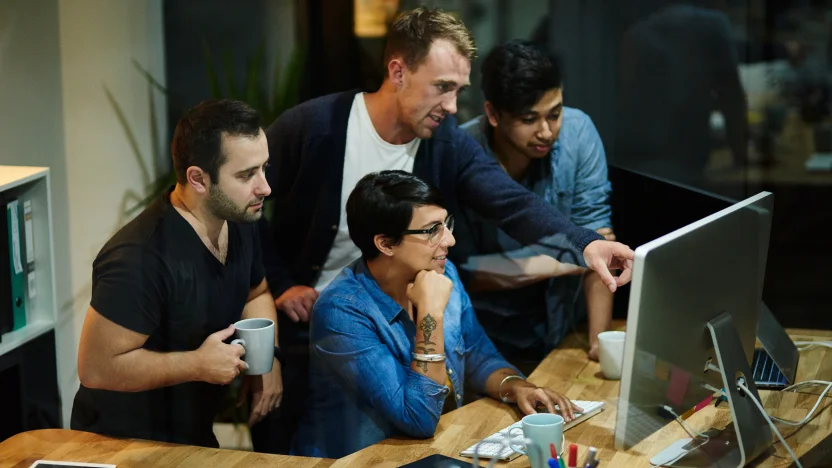
AI-driven sales forecasting and market analysis in the automotive industry
ZBrain provides an advanced solution for sales forecasting and market analysis, transforming processes and empowering automotive businesses to make informed decisions efficiently. By sourcing data from various channels, encompassing sales records, market trends, and customer behavior, ZBrain seamlessly integrates this information into a centralized database for comprehensive analysis. Before the assessment, ZBrain initiates automated Exploratory Data Analysis (EDA) to comprehend data structure, identifying crucial elements like missing values, outliers, correlations, and patterns vital for supplier evaluation. Utilizing advanced techniques during EDA, ZBrain converts data into numerical representations, facilitating effective analysis of relationships and patterns. Upon user queries for sales forecasting or market analysis, ZBrain retrieves relevant data based on specifications, guiding the chosen Language Model (LLM) to generate precise reports. Post LLM report generation, ZBrain parses to sift out irrelevant information, meticulously structuring the data to align with desired formats and guidelines.
DeepSurge’s AI development services for demand forecasting
At DeepSurge, we specialize in developing sophisticated AI solutions tailored to enhance demand forecasting for businesses across various industries. Our strategic AI/ML consulting empowers companies to leverage artificial intelligence to predict market demands more accurately, optimize inventory management, and improve supply chain efficiency.
Our proficiency in developing Proof of Concepts (PoCs) and Minimum Viable Products (MVPs) allows businesses to test the real-world impact of AI tools on demand forecasting, ensuring that solutions are both effective and specifically designed to meet the unique challenges of their market sectors.
Our expertise in generative AI transforms complex data analysis tasks, automating the integration and interpretation of large datasets to free up resources for strategic decision-making.
By fine-tuning large language models to understand industry-specific trends and consumer interactions, LeewayHertz enhances the precision and reliability of demand forecasts, ensuring they align with real-world market dynamics.
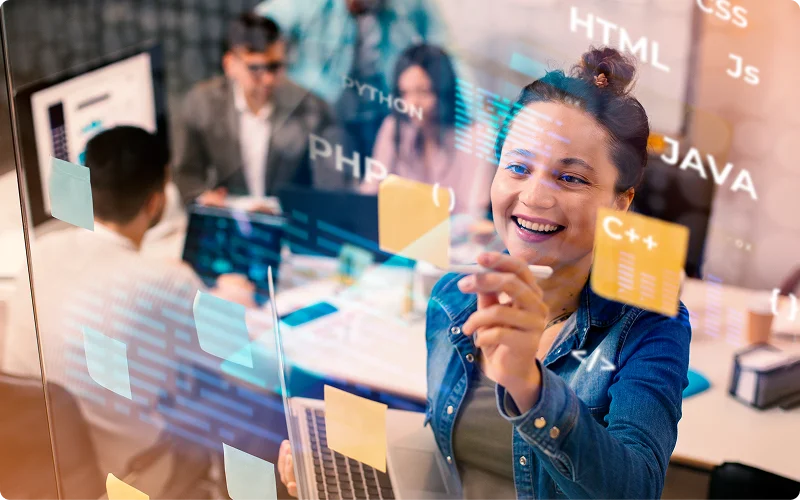
Our AI solutions development expertise
AI solutions development for demand forecasting typically involves creating systems that predict future market demands based on historical data, current market trends, and external factors such as consumer behavior patterns. These solutions integrate key components such as advanced machine learning algorithms and data aggregation technologies, which analyze data from diverse sources like sales records, customer feedback, and market research. This comprehensive data foundation supports predictive analytics capabilities, enabling businesses to anticipate demand fluctuations and plan accordingly. Additionally, these algorithms continuously learn and adapt to new information, ensuring that forecasting models remain accurate. Key areas covered include inventory optimization, production planning, and sales forecasting. Overall, AI solutions in demand forecasting aim to enhance operational efficiency, reduce excess inventory costs, and improve customer satisfaction by ensuring product availability.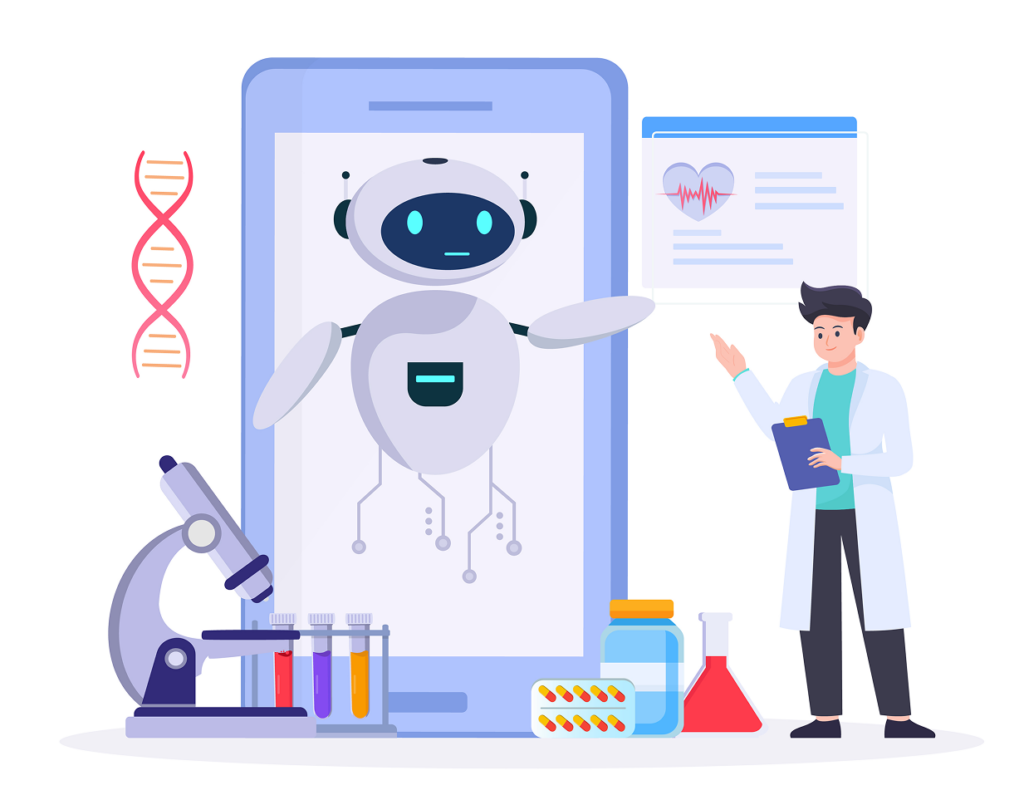
AI agent/copilot development for demand forecasting
DeepSurge builds custom AI agents and copilots that enhance various aspects of demand forecasting, enabling organizations to save time and resources while facilitating more precise and timely decision-making. Here’s how they help:
Advanced demand prediction
- Analyze complex patterns in historical sales data and accurately predict future demand.
- Integrate external data such as market trends, seasonal factors to refine demand predictions.
- Provide real-time updates to demand forecasts, allowing businesses to react quickly to market changes.
Inventory and supply chain optimization
- Optimize inventory levels based on predicted demand, reducing the risk of overstocking or stockouts.
- Automate the alignment of production schedules and supply chain logistics with forecasted demand to improve operational efficiency.
- Enhance supplier management by forecasting demand for raw materials and components, ensuring timely procurement.
Sales and marketing strategy alignment
- Align marketing and promotional strategies with demand forecasts to maximize sales potential.
- Analyze customer purchase behaviors and preferences to target marketing efforts more effectively.
- Optimize pricing strategies dynamically in response to forecasted changes in demand.
Risk management and scenario planning
- Implement scenario planning tools to evaluate the impact of various demand scenarios and prepare contingency plans.
- Identify potential risks in demand fluctuations and develop mitigation strategies.
- Enhance decision-making under uncertainty by providing detailed risk assessments and probability analyses.
Real-time analytics and insights
- Provide stakeholders with real-time insights into demand trends and forecasting accuracy.
- Enable dashboard visualizations of outcomes to facilitate easy interpretation and quicker decision-making.
- Automate the generation of detailed reports on forecast performance, highlighting areas for improvement.
AI-powered demand forecasting vs traditional approaches
Aspect | AI-powered demand forecasting | Traditional approaches |
---|---|---|
Data handling | Can handle large and diverse datasets, including unstructured data such as social media, online activity. | Typically relies on structured historical data and may struggle with diverse or unstructured data. |
Accuracy | Generally has the potential for higher accuracy due to advanced algorithms, machine learning, and pattern recognition capabilities. | Accuracy may vary and is heavily dependent on the quality of historical data and the chosen forecasting methods. |
Adaptability | Can adapt to changing patterns and trends in real-time, making it more responsive to market dynamics. | Often requires manual adjustments and may struggle to adapt quickly to rapidly changing market conditions. |
Forecasting speed | Can forecast faster, allowing for near-real-time updates and quick decision-making. | May take longer to generate forecasts, especially when dealing with large datasets and complex models. |
Human Intervention | Can operate autonomously, reducing the need for extensive manual intervention once the model is trained. | Often requires regular manual adjustments and human expertise to fine-tune forecasts and address anomalies. |
Scalability | Scales well with increasing data and complexity, making it suitable for large-scale operations. | May face challenges in scaling, especially when dealing with substantial increases in data volume and complexity. |
Cost efficiency | Initial implementation costs may be higher, but over time, AI-powered solutions can be cost savings by automating processes. | Initial implementation costs may be lower, but ongoing manual interventions and adjustments can increase operational costs. |
Customization and flexibility | Offers a high degree of customization and flexibility, allowing businesses to tailor models to their specific needs. | Limited flexibility and may rely on standardized models that may not fit the unique characteristics of certain industries or products. |
Benefits of AI-based demand forecasting
In an era of rapid market changes and looming uncertainties, businesses increasingly recognize the need for more sophisticated demand forecasting methods. Though historically reliable, traditional methods often fall short in adaptability and responsiveness. AI and machine learning offer a robust alternative. Let’s examine the tangible benefits that AI in demand forecasting brings to the table:
Improved accuracy
AI algorithms can analyze large datasets and historical patterns more efficiently than traditional methods. This leads to more accurate demand predictions, reducing forecasting errors and improving overall accuracy.
Real-time insights
AI enables real-time analysis of various factors affecting demand, such as market trends, social media mentions. This provides businesses with up-to-the-minute insights, allowing them to respond quickly to changing market conditions.
Enhanced scalability
AI systems can handle large volumes of data, making them scalable for businesses with varying sizes and complexities. As the amount of data increases, AI-based forecasting models can adapt and continue to provide reliable predictions.
Customization and flexibility
AI models can be customized to consider specific industry nuances, seasonality, and other factors that impact demand. This flexibility allows businesses to tailor forecasting models to their unique requirements and adapt to changing market dynamics.
Reduced lead times
AI-based demand forecasting can significantly reduce lead times in decision-making processes. With faster and more accurate predictions, businesses can streamline their supply chain management, reduce stockouts or excess inventory, and improve overall efficiency.
Cost savings
By optimizing inventory levels and minimizing stockouts or overstocks, businesses can achieve cost savings. AI-based forecasting helps in aligning production and supply chain activities with actual demand, reducing carrying costs and avoiding lost sales opportunities.
Improved customer satisfaction
Accurate demand forecasting ensures that products are available when customers want them. This leads to improved customer satisfaction, as businesses can better meet customer expectations and avoid scenarios where popular products are out of stock.
Risk mitigation
AI models can identify potential risks and uncertainties in demand, helping businesses proactively plan for and mitigate these risks. This includes responding to external factors such as economic downturns, natural disasters, or changes in consumer behavior.
Data-driven decision-making
AI leverages data-driven insights to support decision-making processes. This allows businesses to make informed and strategic decisions based on the analysis of historical and real-time data rather than relying solely on intuition or guesswork.
Continuous learning and adaptation
AI systems can continuously learn from new data, adapting their models to changing market conditions. This ongoing learning process ensures that forecasting models remain relevant and effective over time.
How is AI influencing demand forecasting?
Demand forecasting has always been pivotal for businesses to anticipate market changes and strategize accordingly. The recent leaps in machine learning and artificial intelligence have only intensified its importance. The emergence of significant AI models like GPT underscores the vast potential of AI in transforming traditional processes, and demand forecasting is no exception. The unique selling points of AI in demand forecasting hinge on three pillars: dimensionality, scalability, and the feedback loop.
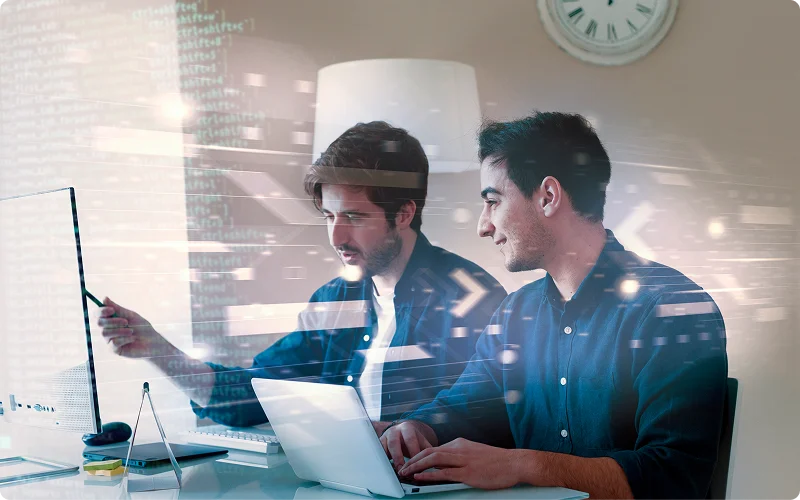
Dimensionality enhances prediction depth
Traditional statistical models mainly use past sales data as their primary source of information. In contrast, AI and machine learning models, especially those using deep learning neural networks, can handle and benefit from a vast number of variables or “features”. The more variables they can analyze, the better their predictions tend to be. This ability allows AI models to consider unconventional types of data that traditional models might overlook. A notable example provided is the use of word embeddings. In this context, word embeddings are a way to convert words or phrases into numeric data that the model can understand and analyze. For example, the phrase “demand forecasting” can be represented by an array of numbers, which might consist of over a thousand individual values. This numeric representation can be used by models like GPT to understand and process information in ways that traditional models can’t.
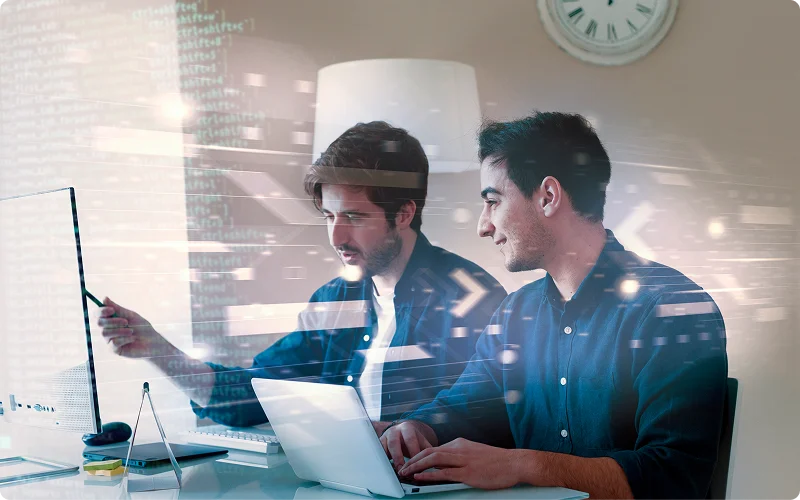
The feedback loop fosters continuous improvement
The lynchpin for enhanced forecasting accuracy is the continuous feedback loop, where forecast models undergo frequent adjustments based on actual sales and customer behaviors. Over time, as models acclimatize to market shifts, forecasts become even more precise. AI supercharges this feedback loop. By autonomously processing vast data streams, AI can pinpoint trends and patterns that might elude human analysts. Such AI-augmented feedback loops enable businesses to construct agile demand forecasting systems, always attuned to the market’s pulse. AI’s imprint on demand forecasting is undeniable. AI reshapes how businesses anticipate market dynamics through its unparalleled dimensionality, scalability, and enriched feedback loop.
Endnote
The integration of AI in demand forecasting is transforming industries worldwide. AI’s ability to decipher patterns, adapt to changing markets, and provide deep insights is redefining forecasting. With the rapid adoption of AI-driven demand forecasting tools by businesses, it is clear that this technology is becoming the industry standard. Future advancements will bring even more sophisticated AI algorithms, real-time data integration, and enhanced predictive analytics, further shaping and influencing market trends. For businesses, keeping up with AI trends in demand forecasting is essential for future success. AI’s role in demand forecasting is not just a trend; it is crucial for leading the future market. Embracing it has become both strategic and necessary for businesses.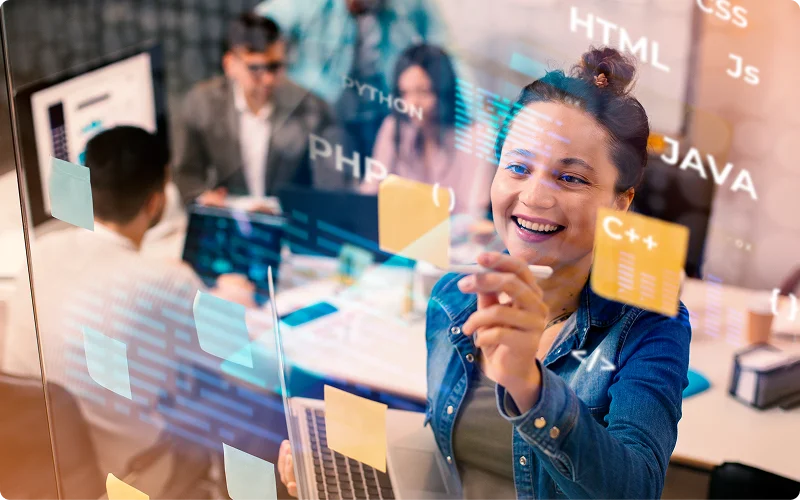
Insights
AI in inventory management: Redefining inventory control for…
AI redefines inventory management, streamlining operations, predicting demand, and optimizing stock control with unrivaled precision and efficiency.
AI in trend analysis: Predicting the pulse of…
In today’s fast-paced, data-driven world, deciphering patterns and trends is more crucial than ever, serving as a compass for organizations and individuals…
A guide to computer vision: Techniques, operational mechanics,…
Computer vision is a field of artificial intelligence that enables computers and systems to derive meaningful insights from digital images, videos, and…