AI in medicine: Applications, benefits, architecture, and future trends
The field of medicine is evolving with the integration of Artificial Intelligence (AI), which is increasingly shaping how medical processes are conducted and managed. AI is proving to be a transformative force, addressing longstanding challenges and introducing new efficiencies. The global AI in healthcare market was valued at USD 15.1 billion in 2022 and is projected to reach approximately USD 187.95 billion by 2030, growing at a compound annual growth rate (CAGR) of 37% during this period. This significant growth underscores the expanding role of AI in medicine. Contact Us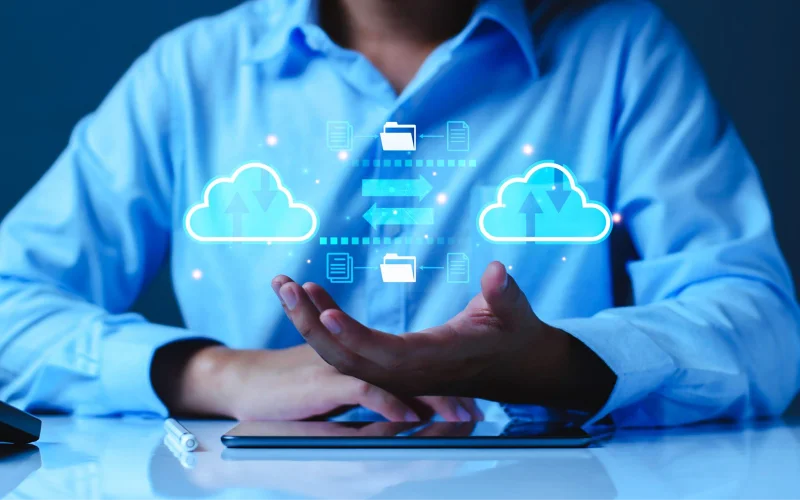
AI’s capabilities extend beyond improving efficiency and precision. For example, AI helps manage and analyze complex datasets, which accelerates drug discovery and development processes. This advancement is crucial for pharmaceutical and biotechnology companies, which face high costs and lengthy development timelines. By utilizing AI, these companies can streamline research and bring new treatments to market more quickly.
Additionally, AI enhances patient care by providing personalized treatment recommendations based on comprehensive health data. This capability addresses challenges related to patient engagement and adherence, enabling healthcare providers to offer more tailored and effective care.
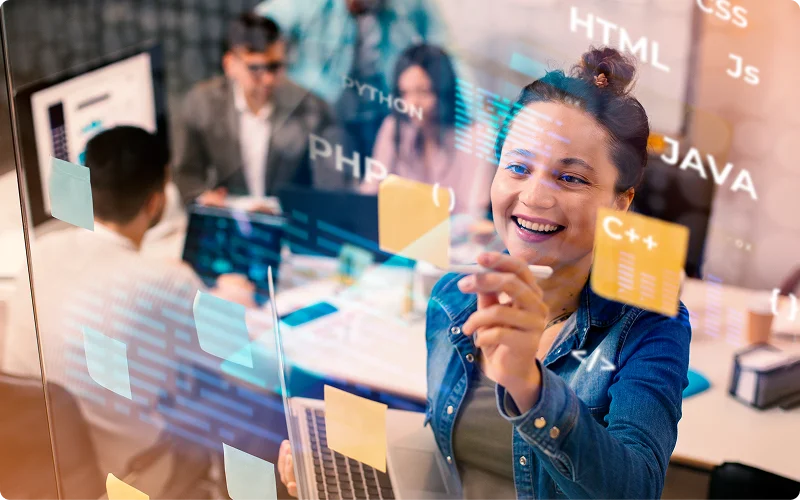
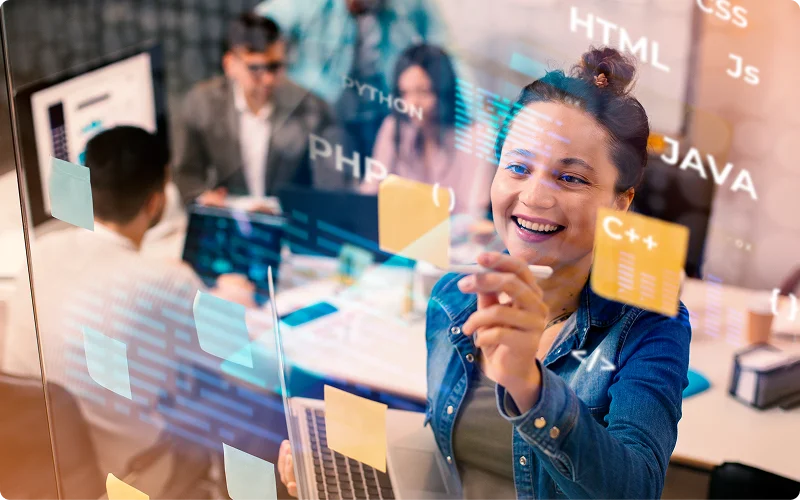
This article explores how AI is reshaping processes in medicine, starting with the challenges faced by companies in the sector and how AI addresses these issues throughout the medicine manufacturing lifecycle. It will delve into the workings of AI in medical practices, highlighting key applications and their benefits. The discussion will cover the diverse roles of AI technologies, offer guidelines for successful implementation, and examine future trends and possibilities. Additionally, the article will address the ethical considerations associated with using AI for medicine, providing a comprehensive overview of AI’s impact on the field.
Challenges faced by companies in the medicine sector
The limitations of traditional methods in the field of medicine pose significant challenges for companies operating in this domain. Despite technological advancements improving healthcare, these challenges persist, emphasizing the need for innovation. Here are some issues faced by companies in medicine when relying on traditional methods:
Limited efficiency
interoperability issues
Scalability challenges
Market competition
Regulatory hurdles
Global healthcare policies
Drug pricing
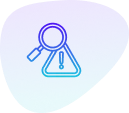
Limited efficiency and productivity
Manual processes, paperwork, and time-consuming procedures in traditional methods can lead to operational inefficiencies, hindering the overall productivity of medical companies.
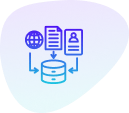
Data silos and interoperability issues
Traditional systems may result in isolated databases, creating data silos that hinder effective communication. Interoperability challenges make seamless sharing of patient data difficult among different entities in the field of medicine.
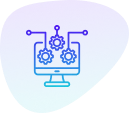
Error-prone processes
Manual data entry and reliance on paper-based records increase the risk of errors in medical documentation, potentially impacting patient safety and the quality of care provided by medical companies.
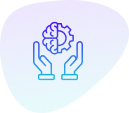
Limited access to patient data
Restrictive access to patient information in traditional methods makes it challenging for healthcare professionals to obtain a comprehensive view of a patient’s medical history, hampering informed decision-making.
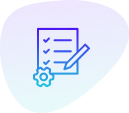
Security and privacy concerns
Weaker security measures in paper records and older electronic systems make them more susceptible to data breaches. Securing privacy and safeguarding the security of patient information is of paramount importance, and traditional approaches may fall short of contemporary standards.
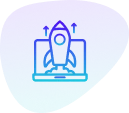
Resource intensiveness
Traditional healthcare methods demand significant resources, including time, manpower, and physical storage space. This resource intensiveness can strain budgets and limit the scalability of services for companies in the medical sector.
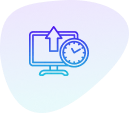
Lack of real-time information
Traditional methods may not provide real-time access to critical information. In the medical field, immediate access to patient data and test results is crucial, especially during emergencies.
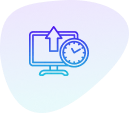
Inefficiencies in communication
Traditional methods may not provide real-time access to critical information. In the medical field, immediate access to patient data and test results is crucial, especially during emergencies.
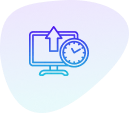
Scalability challenges
Adapting to the increasing demands of a growing population or evolving healthcare environment poses challenges for companies relying on traditional healthcare methods. Scalability issues can limit their ability to adjust to evolving needs.
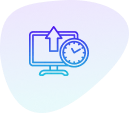
Regulatory hurdles
Pharmaceutical companies must navigate complex regulatory environments, which vary across countries. Compliance with stringent regulations for drug approval and safety, such as those set by the FDA, EMA, and other regulatory bodies, is both critical and challenging.
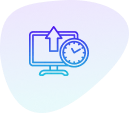
High research and development costs
Developing new medicines is a costly and time-consuming process with a high risk of failure. The extensive research, clinical trials, and development phases require substantial investment.
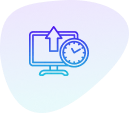
Intellectual property challenges
Protecting intellectual property rights, including patents for new drugs, is essential. However, dealing with patent expirations and generic competition can be challenging in sustaining profitability.
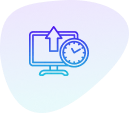
Market competition
Intense competition, both from established pharmaceutical giants and emerging biotech firms, pressures companies to continually innovate and bring new drugs to market swiftly.
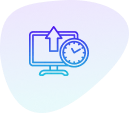
Drug pricing and accessibility
Balancing the pricing of medicines to recover R&D costs while ensuring affordability and accessibility for patients is a significant ethical and financial challenge.
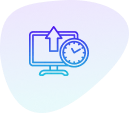
Supply chain complexities
Managing a global supply chain for pharmaceuticals, which includes sourcing raw materials and ensuring timely distribution of medicines, is complex and can be disrupted by geopolitical or global health events.
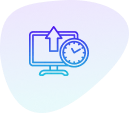
Adverse drug reactions and safety monitoring
Ensuring the safety of medicines and monitoring for adverse drug reactions post-market is crucial. This involves ongoing drug safety monitoring and can impact a company’s reputation and legal standing.
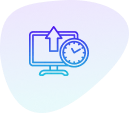
Clinical trial recruitment and efficiency
Recruiting suitable participants for clinical trials and ensuring the trials are conducted efficiently and ethically is a major challenge, directly impacting the drug development timeline.
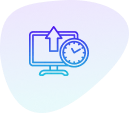
Global healthcare policies
Adapting to different healthcare policies and reimbursement models across countries affects market strategies and access to medicines.
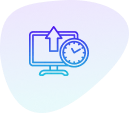
Public trust and image
Maintaining public trust, especially in the wake of controversies or in the face of skepticism about drug efficacy and safety, is crucial for pharmaceutical companies.
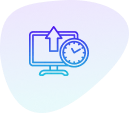
Talent acquisition and retention
The competitive market makes attracting and retaining skilled biotechnology and pharmaceutical research professionals a constant challenge.
How AI addresses challenges across the medicine manufacturing lifecycle
The process of medicine manufacturing involves several stages, from drug discovery to production and distribution. Each stage presents unique challenges, and the integration of AI can overcome these obstacles. Here are some possible challenges in each stage of medicine manufacturing and how AI can help address them:
Discovery and preclinical research
High failure rates
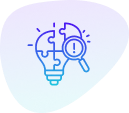
Challenge
Many compounds fail to show promise for further development.
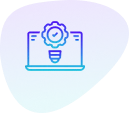
Solution
AI algorithms analyze vast datasets, identifying potential drug candidates with higher success probabilities. Machine learning models can predict compound efficacy and prioritize those with a greater likelihood of advancing to further stages, reducing the overall failure rate.
Resource intensiveness
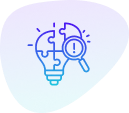
Challenge
Requires significant time and financial resources.
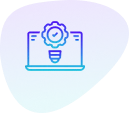
Solution
AI accelerates the drug discovery process by efficiently analyzing data, reducing the time and resources needed. By automating data analysis and optimizing experimental designs, AI mitigates resource-intensive aspects, making drug discovery more cost-effective.
Ethical considerations
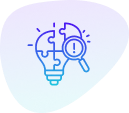
Challenge
Involves ethical concerns, particularly in animal testing during preclinical trials.
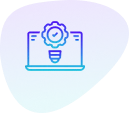
Solution
AI enables the development of in silico models that simulate biological processes, reducing reliance on animal testing. This contributes to more ethical preclinical research by minimizing the need for animal experimentation while still providing valuable insights.
Clinical trials
Patient recruitment
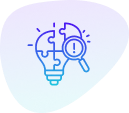
Challenge
Difficulty in recruiting suitable participants.
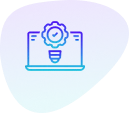
Solution
AI algorithms analyze patient data to identify potential participants who meet specific criteria, improving the efficiency of recruitment. Natural language processing can also assist in extracting relevant information from electronic health records to identify eligible candidates.
Compliance and retention
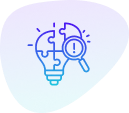
Challenge
Ensuring participant compliance and retention throughout the trial.
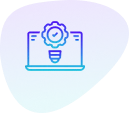
Solution
AI-driven monitoring systems provide real-time data on patient compliance and potential dropout risks. Predictive analytics can identify factors contributing to non-compliance, allowing for proactive interventions to enhance participant retention.
High costs and time consumption
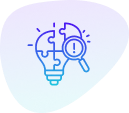
Challenge
Clinical trials are expensive and time-consuming.
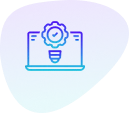
Solution
AI optimizes trial designs, reducing the duration and costs. Predictive modeling helps identify optimal sample sizes, endpoint selection, and protocol adjustments, contributing to more efficient and cost-effective clinical trials.
Regulatory hurdles
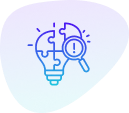
Challenge
Adhering to stringent regulatory requirements for trials.
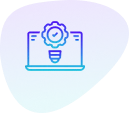
Solution
AI assists in data analysis and interpretation for regulatory submissions, ensuring compliance. Natural language processing aids in parsing complex regulatory documents, facilitating faster and more accurate regulatory approval processes.
Regulatory approval
Data requirements
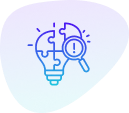
Challenge
Comprehensive data is needed to demonstrate safety and efficacy.
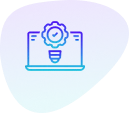
Solution
AI supports the analysis of extensive datasets, extracting relevant information for regulatory submissions. Machine learning models enhance data interpretation, providing a comprehensive view of the safety and efficacy parameters required for regulatory approval.
Uncertain outcomes
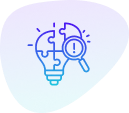
Challenge
Potential for rejection or requests for additional data.
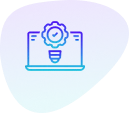
Solution
AI-driven predictive modeling can anticipate potential regulatory concerns, allowing companies to address them proactively. This minimizes the risk of rejection and facilitates a more transparent and collaborative interaction with regulatory authorities.
Varied international standards
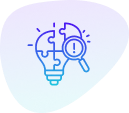
Challenge
Differing regulatory standards in different countries.
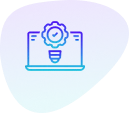
Solution
AI assists in navigating diverse international regulatory landscapes by providing insights into regional requirements. This ensures that regulatory submissions align with the specific standards of each country, facilitating global market access.
Manufacturing and production
Scale-up challenges
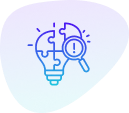
Challenge
Difficulty in scaling up from lab to large-scale production.
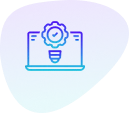
Solution
AI technologies, such as machine learning, predict and optimize manufacturing processes during scale-up. Predictive maintenance models reduce downtime, ensuring a smooth transition from lab-scale to large-scale production.
Quality control
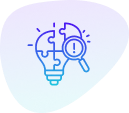
Challenge
Maintaining consistent quality and adhering to regulations.
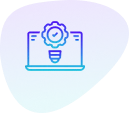
Solution
AI, integrated with sensors and machine learning, enhances real-time quality control by monitoring production processes. This minimizes deviations, ensuring adherence to regulations and consistent product quality.
Supply chain management
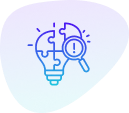
Challenge
Ensuring reliable raw material supply and efficient distribution.
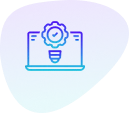
Solution
AI-driven predictive analytics optimize supply chain management by predicting demand, managing inventory levels, and identifying potential risks. Blockchain technology enhances traceability and security, ensuring a reliable and efficient supply chain.
Compounding and granulation
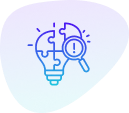
Challenge
Achieving consistency in the compounding and granulation processes.
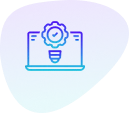
Solution
AI-based control systems can monitor and adjust manufacturing parameters in real-time, ensuring consistency and minimizing variations in the final product.
Tablet compression or capsule filling
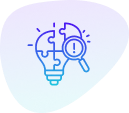
Challenge
Ensuring accurate and efficient tablet compression or capsule filling.
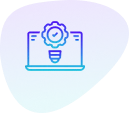
Solution
AI-powered robotics and automation systems can optimize the tablet compression or capsule filling processes, ensuring precision and efficiency.
Packaging
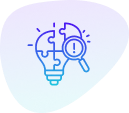
Challenge
Ensuring proper packaging and labeling compliance.
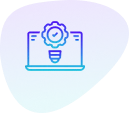
Solution
AI can assist in quality control during packaging, ensuring that products are correctly labeled and packaged according to regulatory standards.
Batch release
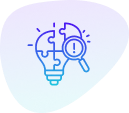
Challenge
Timely and accurate batch release decisions.
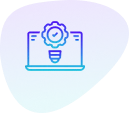
Solution
AI can assist in making batch release decisions by providing real-time analysis of quality control data, ensuring compliance with specifications.
Marketing and distribution
Market access
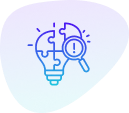
Challenge
Gaining access to and acceptance in the market.
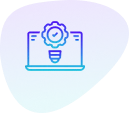
Solution
AI analyzes market trends, patient demographics, and competitor strategies to provide insights for effective market access planning. Predictive analytics helps understand market dynamics, facilitating successful market entry.
Competition
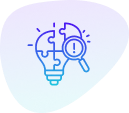
Challenge
Facing competition from other drugs and generic medicines.
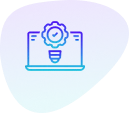
Solution
AI analyzes market competition, predicts competitor strategies, and identifies market gaps. Machine learning algorithms provide recommendations for pricing strategies and market positioning, enabling effective competition management.
Pricing and reimbursement
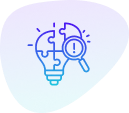
Challenge
Setting appropriate prices and securing reimbursement from healthcare payers.
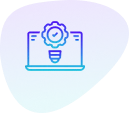
Solution
AI-driven analytics assess market dynamics, healthcare policies, and payer preferences to optimize pricing strategies. Predictive modeling assists in anticipating reimbursement challenges and optimizing negotiations with payers for fair pricing and market access.
Post-marketing surveillance
Detecting adverse effects
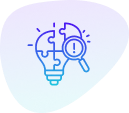
Challenge
Identifying and managing rare or long-term adverse effects.
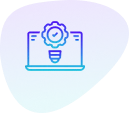
Solution
AI scrutinizes real-world data, encompassing electronic health records and social media, to pinpoint potential safety issues and adverse events. This facilitates faster response to emerging issues and improves post-market surveillance.
Compliance with reporting requirements
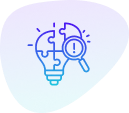
Challenge
Mandatory reporting of adverse effects.
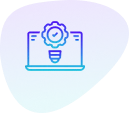
Solution
AI streamlines the reporting process by automating the identification and documentation of adverse events, ensuring timely and accurate compliance with reporting requirements.
Ongoing regulatory compliance
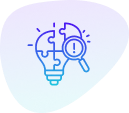
Challenge
Continuing to meet regulatory standards.
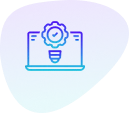
Solution
AI-driven systems provide continuous monitoring of regulatory requirements, ensuring ongoing compliance. Predictive modeling can anticipate changes in regulations, allowing companies to adapt their processes accordingly.
Patent expiry and generic production
Loss of exclusivity
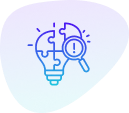
Challenge
Revenue loss when generic versions enter the market.
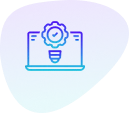
Solution
AI aids in forecasting the impact of patent expiry by analyzing market trends, competitor activities, and historical data. Predictive modeling helps develop strategies to mitigate revenue loss, such as exploring new indications, formulations, or partnerships.
Market competition
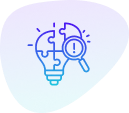
Challenge
Maintaining market share against generic competitors.
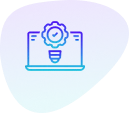
Solution
AI continuously monitors the market and competitive landscape, providing real-time insights. Machine learning algorithms identify opportunities to differentiate products, optimize marketing strategies, and enhance customer engagement, helping companies maintain market share amid generic competition.
How does AI for medical practices work?
AI is transforming the field of medicine by leveraging advanced analytics, machine learning, and deep learning to enhance diagnostic accuracy, personalize treatment plans, and improve patient outcomes. Utilizing advanced Large Language Models (LLMs) within a structured architecture and integrating them with the healthcare organization’s proprietary knowledge bases enables the processing of vast amounts of medical data to identify trends, diagnose diseases earlier, and recommend the most effective treatments.
The structured architecture of the AI-powered medical system integrates multiple components and operates as follows:
Data sources
Effective medical practices rely on diverse and comprehensive data inputs, including:
- Patient health records: Detailed histories, including previous diagnoses, treatments, and outcomes, provide a comprehensive view of each patient’s health.
- Medical imaging data:High-resolution images from MRIs, CT scans, and X-rays for diagnosing and monitoring diseases.
- Genomic data: Information on genetic markers that influence the risk of certain diseases and treatment responses.
- Clinical trial data: Results from past and ongoing clinical trials that help understand treatments’ effectiveness and side effects.
- Real-time health monitoring data: Data from wearable devices that monitor patients’ vital signs, like heart rate, blood pressure, and glucose levels.
- Patient feedback and interaction data: Input from patient interactions via virtual health platforms helps assess treatment effectiveness and patient satisfaction.
Data pipelines
The data gathered from the above sources undergoes processing through data pipelines. These pipelines handle data ingestion, cleaning, and structuring tasks, ensuring the data is prepared for subsequent analysis.
Embedding model
An embedding model converts textual data into numerical representations called vectors that AI models can understand. Popular models from providers like OpenAI, Google, and Cohere are utilized.
Vector database
The resulting vectors are stored in a vector database, facilitating efficient querying and retrieval. Prominent examples of vector databases include Pinecone, Weaviate, and PGvector.
APIs and plugins
APIs and plugins like Serp, Zapier, and Wolfram play a key role by connecting different components and enabling additional functionalities, such as accessing extra data, integrating with healthcare platforms or performing specific tasks with ease.
Orchestration layer
The orchestrating layer is critical in managing the workflow. ZBrain is an example of this layer that simplifies prompt chaining, manages interactions with external APIs by determining when API calls are required, retrieves contextual data from vector databases, and maintains memory across multiple LLM calls. Ultimately, this layer generates a prompt or series of prompts submitted to a language model for processing. The role of this layer is to orchestrate the flow of data and tasks, ensuring seamless coordination across all components within the architecture.
Query execution
The data retrieval and generation process commences when the user submits a query to the medical management app. This query can be about diagnoses, treatment options, or patient’s real-time details and conditions.
LLM processing
Once received, the app transmits the query to the orchestration layer. This layer retrieves relevant data from the vector database and LLM cache and sends it to the appropriate LLM for processing. The choice of LLM depends on the nature of the query.
Output
The LLM generates an output based on the query and the data it receives. This output can come in various forms, such as potential diagnoses, treatment recommendations, and predictive health outcomes.
Healthcare application
The validated output is presented to the user through the healthcare application. This application organizes and presents the data, analysis, and insights in a user-friendly format tailored for medical professionals or administrators.
Medical management app
This specialized app delivers AI-generated insights in an accessible format, enabling doctors and healthcare professionals to make informed decisions quickly and tailor their care to individual patient needs.
Feedback loop
User feedback on the LLM’s output is another vital aspect of this architecture. This feedback loop is instrumental in progressively refining the accuracy and relevance of the LLM’s output over time.
Feedback loop
User feedback on the AI-generated output is crucial in this architecture, which helps refine accuracy and relevance. This feedback loop improves the model’s performance over time.
Agent
AI agents enter this process to address complex problems, interact with the external environment, and enhance learning through post-deployment experiences. They achieve this by employing advanced reasoning/planning, strategic tool utilization, and leveraging memory, recursion, and self-reflection.
LLM cache
Tools like Redis, SQLite, or GPTCache are used to cache frequently accessed information, accelerating the AI system’s response time.
Logging/LLMOps
Throughout this process, LLM operations (LLMOps) tools like Weights & Biases, MLflow, Helicone, and Prompt Layer assist in logging actions and monitoring performance. This ensures that LLMs operate at their best and continually enhance through feedback loops.
Validation
A validation layer is integrated to verify the accuracy and reliability of the LLM’s output. Tools such as Guardrails, Rebuff, Guidance, and LMQL ensure that the information provided meets the required standards.
LLM APIs and hosting
LLM APIs and hosting platforms are essential for executing crucial tasks and hosting the application. Depending on the requirements, developers can select from LLM APIs, such as those offered by OpenAI and Anthropic or opt for open-source models. Similarly, they can choose hosting platforms from cloud providers like AWS, GCP, Azure, and Coreweave or opt for opinionated clouds like Databricks, Mosaic, and Anyscale. The choice of LLM APIs and cloud hosting platforms depends on the project’s needs.
AI in medicine: Key applications and their benefits
AI has found diverse applications in medicine, transforming various aspects of healthcare delivery and improving patient outcomes. Here are some key applications of AI in health and medicine:
Research and development
AI in protein structure prediction
AI in protein structure prediction involves using advanced algorithms to determine the three-dimensional structures of proteins based on their amino acid sequences. This capability significantly accelerates drug discovery and deepens our understanding of biological processes and diseases. As a crucial application of AI in medical research, predicting protein structures helps identify potential drug targets and design novel therapeutics more efficiently. The ability to model protein structures with high accuracy can lead to breakthroughs in understanding diseases at a molecular level and optimizing drug development, potentially reducing the time and cost associated with traditional methods.
AI-driven literature review and meta-analysis
AI-driven literature review and meta-analysis utilize sophisticated algorithms to automatically analyze and synthesize findings from extensive medical literature. These systems represent a significant advancement in AI-driven medical research, as they can process vast amounts of research articles, extract relevant data and identify trends and correlations that might not be immediately apparent to human researchers. By automating this process, AI enhances the speed and accuracy of literature reviews, supports evidence-based medicine, and helps researchers stay current with the rapidly growing volume of scientific knowledge. This capability ensures that healthcare decisions and research directions are grounded in the most comprehensive and up-to-date evidence available.
In silico clinical trials
In silico clinical trials use AI and computer simulations to model and predict the outcomes of clinical trials without relying solely on human subjects. By creating virtual environments to simulate the effects of new drugs or interventions, these AI models can assess efficacy and safety during the early stages of drug development. This approach not only speeds up the research process but also reduces the ethical and logistical challenges associated with traditional clinical trials. In silico trials enable researchers to refine trial designs, optimize protocols, and identify potential issues before conducting real-world studies, thereby improving the overall efficiency and effectiveness of drug development.
Clinical trial research
AI plays a crucial role in transforming clinical trial research by optimizing various aspects of the trial process. One key application is in the design phase, where AI algorithms analyze vast datasets to identify optimal trial parameters, such as patient demographics, inclusion criteria, and outcome measures. By leveraging machine learning and predictive analytics, AI can predict patient responses to treatments and refine trial protocols, leading to more efficient and cost-effective trials. Additionally, AI helps in identifying suitable candidates for clinical trials by analyzing patient data to match individuals with specific trial criteria, thereby streamlining the patient recruitment process. Furthermore, AI models can predict trial outcomes based on patient characteristics, treatment regimens, and other factors, providing valuable insights for researchers and clinicians. Overall, AI-driven approaches in clinical trial research enhance the efficiency and effectiveness of trials, ultimately accelerating the development of new treatments and therapies for various medical conditions.
Diagnosis and treatment
Diagnostic imaging
AI plays a pivotal role in diagnostic imaging by leveraging advanced algorithms, such as Convolutional Neural Networks (CNNs), to analyze medical images. These algorithms can detect patterns and abnormalities in images like X-rays, MRIs, and CT scans. Computer-aided detection and diagnosis (CAD) systems powered by AI assist radiologists by highlighting potential areas of concern and providing insights that contribute to more accurate and timely diagnoses. This application enhances the efficiency of radiological assessments, potentially leading to earlier detection of diseases.
Clinical Decision Support Systems (CDSS)
CDSS leverages the power of AI to analyze diverse sources of information, including patient data, medical literature, and clinical guidelines. By doing so, they offer evidence-based recommendations to healthcare providers, assisting them in crucial aspects of patient care such as diagnosis, treatment planning, and overall management. These systems act as invaluable tools in the healthcare setting, augmenting the expertise of healthcare professionals by providing them with insights derived from vast amounts of data. With AI-driven CDSS, healthcare providers can make more informed decisions, leading to improved patient outcomes, enhanced efficiency, and, ultimately, better quality of care.
Personalized medicine
Personalized medicine, enabled by AI, involves tailoring treatment plans based on individual patient characteristics and responses. AI scrutinizes varied datasets, encompassing genetic information, to pinpoint the most optimal interventions tailored to a specific patient. This approach aims to maximize treatment efficacy while minimizing adverse effects. By customizing medical interventions to each patient’s unique biological makeup, personalized medicine holds the promise of more precise and targeted healthcare.
Mental health analysis and treatment
AI is transforming traditional approaches by offering innovative tools and interventions in the domain of mental health analysis and treatment. Advanced AI algorithms are being developed to analyze speech patterns, facial expressions, and writing, enabling the detection of mental health issues with unprecedented accuracy. By leveraging natural language processing and sentiment analysis techniques, these tools can sift through vast amounts of data to identify subtle indicators of psychological distress or disorders. Moreover, AI-powered systems can provide cognitive behavioral therapy (CBT) and other evidence-based interventions, offering personalized support to individuals in need. By automating aspects of mental health assessment and therapy, AI enhances accessibility to care, reduces stigma, and complements the efforts of healthcare professionals, ultimately improving outcomes and promoting overall well-being.
Drug discovery and development
In the realm of drug discovery, AI accelerates the identification of potential drug candidates and optimizes the drug development process. Machine learning algorithms analyze vast datasets, including biological and chemical information, to predict how molecules will interact. This predictive capability aids researchers in designing drugs more efficiently, potentially speeding up the development of new therapeutic agents. By leveraging AI in drug discovery, the pharmaceutical industry can explore a broader range of possibilities for treating various diseases.
Drug repurposing
Drug repurposing, also known as drug repositioning or reprofiling, is an innovative application of AI in medicine aimed at identifying new uses for existing drugs beyond their originally intended purposes. Leveraging AI algorithms, researchers can analyze vast datasets of biological and chemical information to uncover potential therapeutic properties of approved drugs for treating different diseases. By repurposing existing drugs, the drug development process can be expedited, as the safety profiles and pharmacokinetics of these drugs are already well-established. Furthermore, drug repurposing offers a cost-effective approach to discovering new treatments, as it bypasses many of the time-consuming and resource-intensive steps typically associated with traditional drug discovery. Overall, AI-driven drug repurposing holds significant promise for accelerating the development of novel therapies and addressing unmet medical needs in a wide range of diseases.
Genomic analysis
Genomic analysis transforms the understanding and treatment of various diseases by deciphering the complexities of the human genome. AI algorithms analyze vast genomic datasets to identify genetic variations, mutations, and biomarkers associated with disease susceptibility, progression, and treatment response. By integrating multi-omics data, including genomics, transcriptomics, proteomics, and metabolomics, AI enables comprehensive analyses that unravel the intricate molecular mechanisms underlying diseases. One significant application of AI in genomic analysis is in the field of precision medicine. AI-driven predictive modeling can assess an individual’s genetic makeup to tailor personalized treatment plans based on their unique genetic profile. This approach maximizes treatment efficacy while minimizing adverse effects, leading to more precise and targeted therapeutic interventions.
Patient engagement and healthcare delivery
Virtual health assistants
AI-driven virtual health assistants, including chatbots, provide a range of services, such as answering patient queries, offering information on medical conditions, and assisting with administrative tasks. These virtual assistants operate 24/7, enhancing accessibility to healthcare information and services. Automating routine tasks allows healthcare professionals to focus more on direct patient care, ultimately improving the patient experience and engagement.
Telemedicine and virtual consultations
AI technologies facilitate telemedicine by enabling virtual healthcare interactions, including video consultations and AI-driven diagnostics. Telemedicine improves access to healthcare services, allowing patients to consult with healthcare providers remotely. AI-driven diagnostics support the interpretation of symptoms, aiding in the provision of timely and efficient healthcare services, especially in situations where in-person visits are challenging or not feasible.
Remote patient monitoring
AI, in conjunction with Internet of Things (IoT) devices and wearables, enables continuous monitoring of patients’ vital signs and health parameters. These connected devices transmit real-time data to AI systems, allowing healthcare providers to remotely track patients’ well-being. This application is particularly valuable for managing chronic conditions and facilitating early intervention in case of health deviations, reducing the need for frequent hospital visits.
AI-driven genetic counseling
Artificial intelligence plays a transformative role in genetic counseling by streamlining various processes through advanced chatbot technology. In this application, AI-driven chatbots are employed to manage and enhance the genetic counseling experience. These chatbots facilitate the collection of essential patient information, including personal and family medical histories, through secure and interactive virtual conversations. By automating the intake process, AI chatbots help reduce the administrative burden on healthcare professionals and ensure that comprehensive data is collected efficiently.
Population health and healthcare systems
Predictive analytics for patient outcomes
AI-powered predictive analytics leverages patient data to forecast health outcomes and assess the risk of hospital readmission. These AI models are instrumental for healthcare providers in identifying high-risk patients. This identification enables proactive interventions, helping to prevent complications and reduce the strain on healthcare resources. Additionally, predictive analytics play a key role in enhancing resource allocation efficiency and in the formulation of personalized care plans tailored to individual patient needs.
Epidemiology and public health
Within the domain of epidemiology and public health, AI functions as a valuable asset for tracking and forecasting disease spread, thus enabling proactive public health interventions. By harnessing sophisticated algorithms, AI can analyze vast datasets sourced from diverse channels such as social media, news reports, and governmental databases. Through data mining and pattern recognition techniques, AI algorithms can identify early indicators of disease outbreaks, track transmission patterns, and predict future trends with remarkable accuracy.
Healthcare fraud detection
Within healthcare fraud detection, AI emerges as a powerful tool capable of mitigating financial losses and preserving the integrity of healthcare systems. Leveraging sophisticated algorithms, AI can scrutinize vast volumes of healthcare data, including billing records, insurance claims, and patient information, to identify patterns indicative of fraudulent behavior. By analyzing subtle anomalies and discrepancies in billing practices, AI algorithms can flag suspicious activities, such as overbilling, upcoding(assigning higher reimbursement codes than justified), and phantom billing schemes(billing for services or patients that don’t exist), which may otherwise go undetected.
Improving healthcare operations and administration
Electronic Health Records (EHR)
AI, particularly Natural Language Processing (NLP), transforms the management of Electronic Health Records (EHR). NLP algorithms can extract meaningful information from unstructured clinical notes, making organizing and accessing patient data easier. For example, NLP can be used to automate coding by extracting relevant information from physician notes or to generate concise summaries of patient records, streamlining documentation and improving information access.
AI for healthcare operations
AI is transforming healthcare operations by streamlining administrative tasks and optimizing workflows. AI-powered systems can analyze appointment schedules, patient flow patterns, and resource availability to optimize appointment scheduling, minimizing wait times for patients and optimizing the utilization of healthcare resources. AI can also automate routine administrative tasks, such as data entry, insurance verification, and appointment reminders, freeing up healthcare professionals to focus on patient care. These efficiencies not only improve the patient experience but also contribute to cost reductions and improved resource allocation within healthcare organizations.
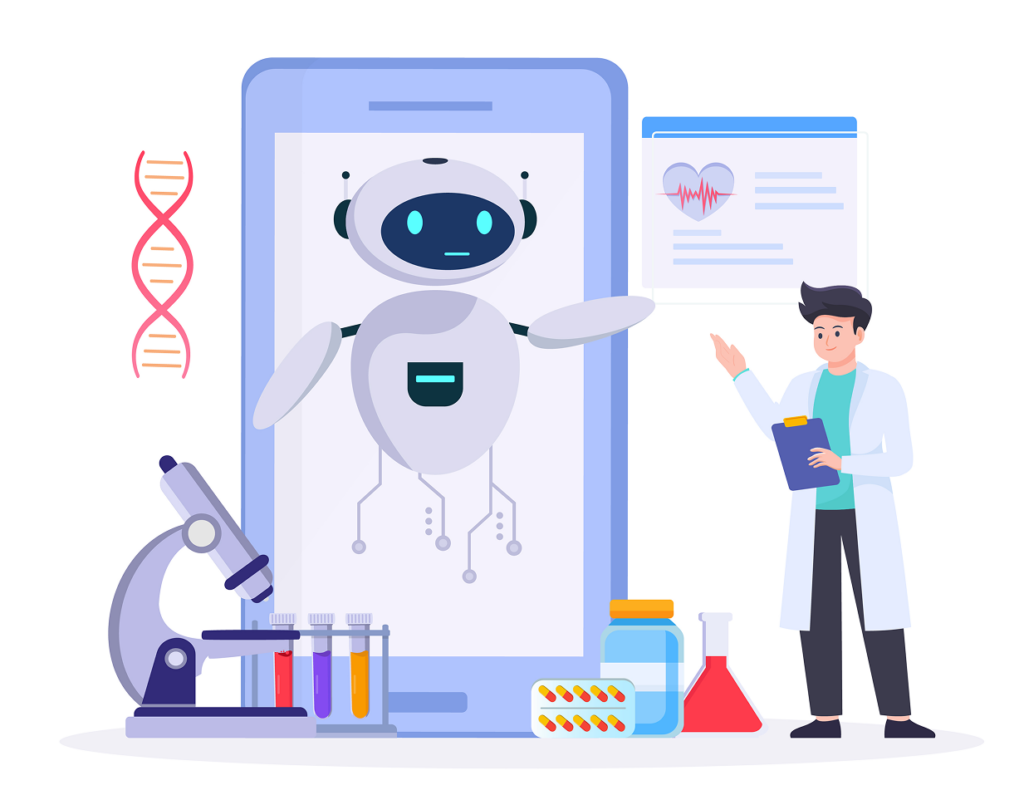
Innovative applications of generative AI in medicine
Generative AI (GenAI) is making significant strides in the field of medicine, bringing a fresh perspective to various applications. Unlike traditional AI models, which are primarily used for analyzing existing data, GenAI focuses on generating new data, insights, and solutions. This capability opens up exciting opportunities across different areas of medicine.
One of the key applications of generative AI in medicine is in drug discovery. By creating novel molecular structures and simulating their interactions, GenAI can propose new drug candidates that may not be evident through conventional methods. GenAI can also design molecules with specific properties, like better bioavailability or reduced side effects, which accelerates the drug development process and opens up possibilities for discovering treatments for complex and rare diseases.
In medical imaging, GenAI enhances the quality and detail of synthetic images, which can be used for training diagnostic models. This can help improve the accuracy of imaging systems and provide more diverse datasets for research and training purposes. For example, GenAI can generate high-resolution images from low-quality scans, aiding in better diagnostic assessments. By generating synthetic images of rare diseases, GenAI can create training datasets for diagnostic models that would otherwise be difficult to obtain, leading to improved diagnostic accuracy for conditions with limited data.
GenAI also plays a role in personalized medicine by generating tailored treatment plans based on a patient’s unique genetic makeup and medical history. For instance, GenAI can analyze a patient’s genome and medical history to suggest alternative treatment pathways or predict their response to specific therapies, offering a more customized approach to patient care.
Another significant application is in the creation of virtual simulations for medical training and education. GenAI can generate realistic, interactive scenarios that allow medical professionals to practice and refine their skills in a controlled environment. This contributes to better training outcomes and prepares practitioners for a wide range of clinical situations. By simulating rare or complex surgical procedures, GenAI can provide valuable training experiences that would be impractical or dangerous in real-world settings, ultimately leading to improved patient outcomes.
How DeepSurge’s generative AI platform streamlines medical processes
DeepSurge’s generative AI platform, ZBrain, is a vital tool helping enhance and streamline various aspects of medical processes within healthcare institutions. By creating custom LLM-based applications tailored to clients’ proprietary medical data, ZBrain optimizes medical workflows, ensuring operational efficiency and elevated patient care. The platform processes diverse medical data types, including patient records and research documents, and utilizes advanced language models like GPT-4, Vicuna, Llama 2, and GPT-NeoX to build context-aware applications that can improve decision-making, deepen insights, and boost overall productivity, all while maintaining strict data privacy standards, making it indispensable for modern medical operations.
This ensures heightened operational efficiency, minimized error rates, and elevated overall quality in medical processes. ZBrain emerges as a versatile solution, offering comprehensive answers to some of the most intricate challenges in the medical industry. Here are a few examples –
AI-driven pharmaceutical pricing and promotion
In the pharmaceutical industry, where competition is fierce and markets are ever-changing, setting the right prices and planning effective promotions are crucial. ZBrain plays a crucial role in helping businesses with pharmaceutical pricing and promotion. ZBrain’s LLM apps provide valuable insights for setting competitive prices and designing impactful promotions in a fraction of the traditional time.
Personalized treatment planning
AI agents analyze patient data to tailor treatment plans to individual patient’s needs and preferences. By considering factors such as genetics, medical history, and lifestyle, these agents can recommend personalized treatment options that maximize efficacy and minimize side effects.
DeepSurge’s AI development services for medicine
At DeepSurge, we craft tailored AI solutions that cater to the unique requirements of healthcare providers and medical facilities. We provide strategic AI/ML consulting that enables healthcare organizations to harness AI for enhanced clinical decision-making, improved patient engagement, and optimized treatment strategies.
Our expertise in developing Proof of Concepts (PoCs) and Minimum Viable Products (MVPs) allows healthcare providers to preview the potential impacts of AI tools in real clinical scenarios, ensuring that the solutions are effective and tailored to the medical sector’s specific needs.
Our work in generative AI also transforms routine tasks like medical report generation, patient data management, insurance claims processing and more, automating these processes to free healthcare professionals for more critical roles.
By fine-tuning large language models to the nuances of medical terminology and patient interactions, LeewayHertz enhances the accuracy and relevance of AI-driven communications and analyses in healthcare.
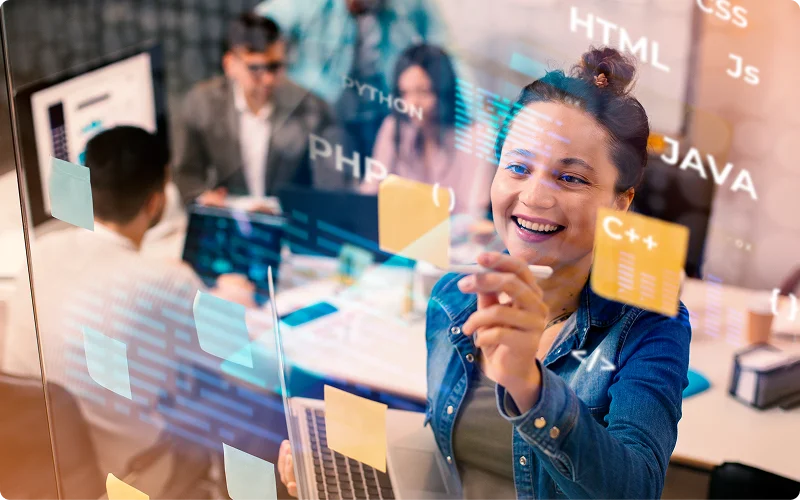
Our AI solutions development expertise
AI solutions development for healthcare typically involves creating systems that enhance clinical decision-making, automate routine tasks, and personalize patient care. These solutions integrate key components such as data aggregation technologies, which compile and analyze medical information from diverse sources like electronic health records (EHRs), medical imaging, and genomic data. This comprehensive data foundation supports predictive analytics capabilities, allowing for the forecasting of disease progression and treatment outcomes that inform clinical decisions. Additionally, machine learning algorithms are employed to tailor treatment plans to individual patient profiles, ensuring that each patient’s unique medical history, risk factors, and preferences are considered. These solutions often cover areas like diagnosis, treatment planning, drug discovery, patient monitoring, and care coordination. Overall, AI solutions in healthcare aim to improve patient outcomes, enhance operational efficiency, and elevate the quality of care delivery.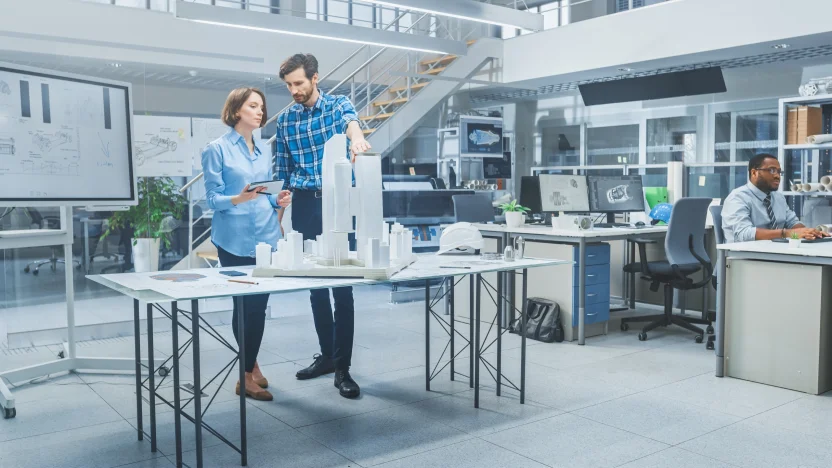
AI agent/copilot development for medicine
AI has found diverse applications in medicine, transforming various aspects of healthcare delivery and improving patient outcomes. Here are some key applications of AI in health and medicine:
Diagnosis & treatment planning
- Analyzing medical images like X-rays, CT scans, and MRIs, aiding in faster and more accurate diagnosis of diseases.
- Analyzing patient data, such as medical history, genetic information, and lifestyle factors and predict the likelihood of developing specific diseases, enabling early intervention and prevention.
- Suggesting personalized treatment plans, including medication choices, dosages, and therapies.
Drug discovery & development
- Analyzing vast amounts of data to identify new potential drug targets, accelerating the discovery of new therapies.
- Assisting in designing efficient and cost-effective clinical trials, speeding up the process of bringing new drugs to market.
- Identifying existing drugs that could be effective for new diseases or conditions.
- Optimizing clinical trials by identifying the most suitable participants and predicting trial outcomes.
Patient management & care
- Tracking patients’ vital signs, medication adherence, and symptoms, enabling early detection of health problems.
- Tailoring treatments and care plans to individual patient needs, improving outcomes and reducing healthcare costs.
- Providing patient education, answering questions, and scheduling appointments reduces the workload of healthcare professionals.
- Delivering personalized educational content and providing ongoing support for patients with chronic conditions.
Administrative & operational processes
- Streamlining the management of patient records, medical billing, and administrative processes, improving efficiency and accuracy.
- Analyzing data to predict patient flow, resource needs, and potential issues allows healthcare organizations to optimize operations and allocate resources effectively.
- Automating repetitive tasks, such as scheduling appointments, ordering tests, and generating reports, freeing healthcare professionals to focus on patient care.
- Automating tedious tasks like coding medical records and processing claims, improving efficiency and reducing errors.
- Analyzing large datasets to generate insights into patient trends, disease patterns, and healthcare system performance.
Treatment and management
- Accelerating drug discovery by analyzing large datasets and identifying potential drug targets.
- Tailoring treatment plans based on individual patient characteristics and predicting drug response.
- Providing doctors with real-time insights and recommendations during patient care, aiding in diagnosis and treatment selection.
- Analyzing patient data like wearable sensor readings to monitor disease progression and alert clinicians to potential issues.
- Analyzing vast amounts of medical literature to identify trends and emerging research areas.
The diverse role of AI technologies in medicine
Several AI technologies are actively utilized in medicine, contributing to advancements in diagnosis, treatment, and overall healthcare delivery. Here are some key AI technologies employed in the field of medicine:
Our AI solutions development expertise
AI solutions development for healthcare typically involves creating systems that enhance clinical decision-making, automate routine tasks, and personalize patient care. These solutions integrate key components such as data aggregation technologies, which compile and analyze medical information from diverse sources like electronic health records (EHRs), medical imaging, and genomic data. This comprehensive data foundation supports predictive analytics capabilities, allowing for the forecasting of disease progression and treatment outcomes that inform clinical decisions. Additionally, machine learning algorithms are employed to tailor treatment plans to individual patient profiles, ensuring that each patient’s unique medical history, risk factors, and preferences are considered. These solutions often cover areas like diagnosis, treatment planning, drug discovery, patient monitoring, and care coordination.
Overall, AI solutions in healthcare aim to improve patient outcomes, enhance operational efficiency, and elevate the quality of care delivery.
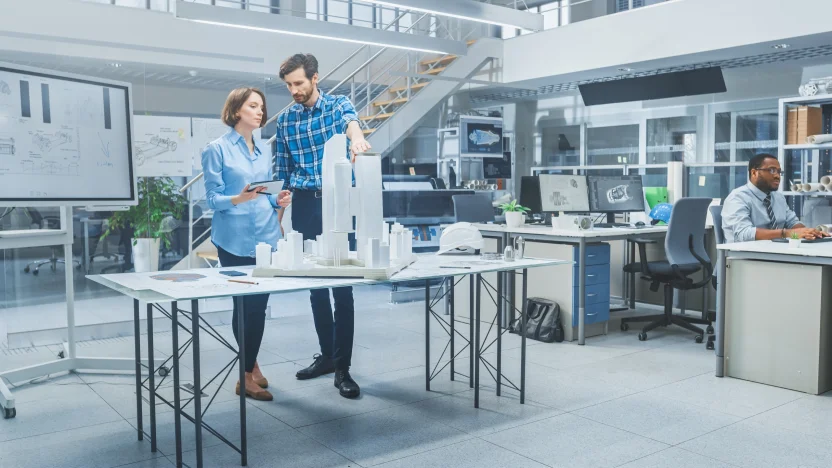
Natural Language Processing (NLP)
NLP has emerged as a transformative force in the medical field, redefining the extraction and interpretation of information from unstructured clinical texts. In medicine, NLP algorithms dissect the complexities of medical narratives, including electronic health records (EHRs), clinical notes, and research papers, to derive meaningful insights. Named Entity Recognition (NER) algorithms are commonly employed to identify and classify medical entities such as diseases, medications, and procedures within the text. Additionally, part-of-speech tagging and syntactic parsing enable the understanding of the grammatical structure of medical documents, aiding in the extraction of relationships between entities. The application of sentiment analysis proves valuable in assessing the emotional tone of patient narratives, contributing to a more comprehensive understanding of the patient experience. As the volume of medical information continues to grow, NLP’s ability to unlock valuable information from textual data enhances decision-making, supports clinical research, and ultimately contributes to more informed and efficient healthcare delivery.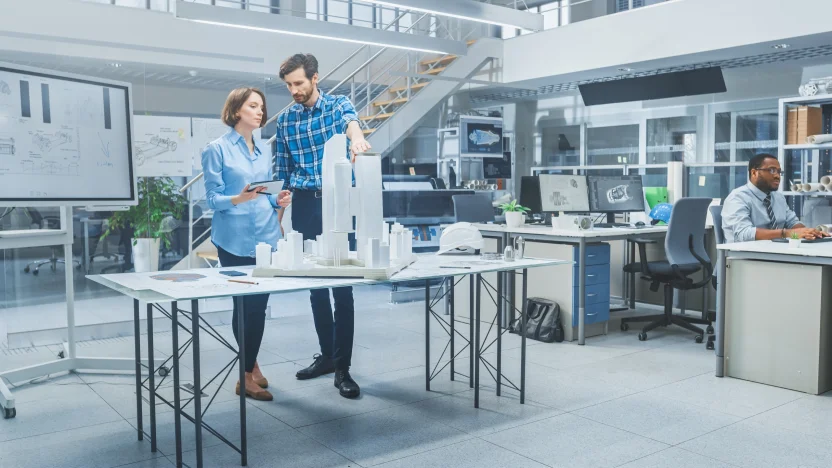
Computer vision
Computer vision has redefined medical imaging and diagnostics, offering advanced capabilities in the analysis of visual data. In medicine, Computer Vision algorithms, particularly Convolutional Neural Networks (CNNs), play a pivotal role in tasks such as image recognition, segmentation, and classification. In radiology, CNNs excel in detecting patterns and anomalies in X-rays, CT scans, and MRIs, providing detailed insights for accurate diagnoses. Image segmentation algorithms enable the precise delineation of organs and structures, facilitating targeted treatment planning. Beyond diagnostics, Computer Vision is applied in surgical navigation systems, where algorithms process real-time images to assist surgeons in navigating complex anatomical structures. Furthermore, Computer Vision contributes to pathology, aiding pathologists in the identification and classification of tissue samples. As the field advances, the synergy between Computer Vision and medical imaging continues to enhance the accuracy and efficiency of diagnostics and treatment planning, propelling the evolution of modern healthcare practices.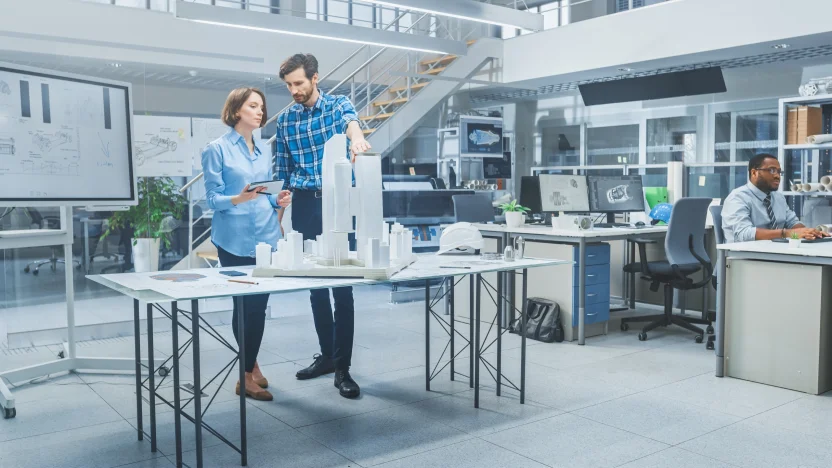
Deep learning
Deep learning has become instrumental in transforming various facets of medicine, particularly in the analysis of complex data. In the realm of medical applications, deep neural networks, specifically Convolutional Neural Networks (CNNs) and Recurrent Neural Networks (RNNs), are widely employed due to their extensive utility. CNNs excel in image-based tasks, enabling intricate pattern recognition in medical imaging, such as identifying abnormalities in X-rays or detecting features in histopathological slides. RNNs, with their sequential learning capabilities, are applied in tasks involving time-series data, such as monitoring patient vital signs and predicting disease progression. Deep Learning models showcase unparalleled performance in personalized medicine by processing vast datasets, including genomics and patient records, to tailor treatment plans based on individual characteristics. The capacity of deep neural networks to autonomously acquire intricate representations from data positions them at the forefront of medical research, diagnostics, and treatment optimization, signifying a paradigm shift in healthcare practices.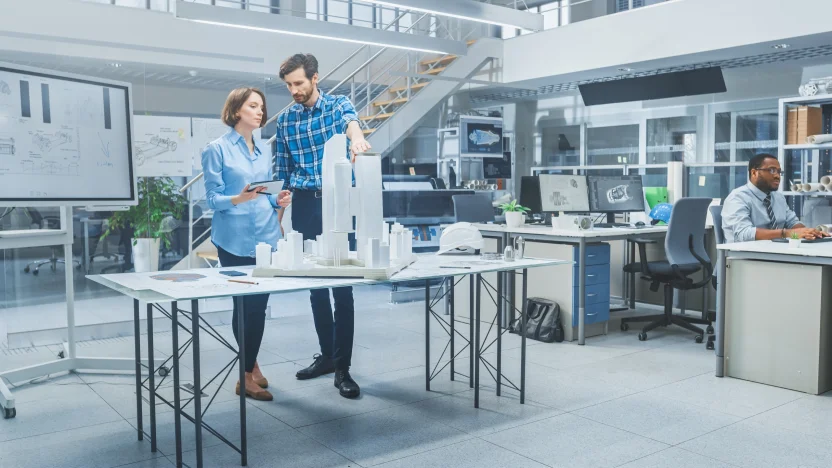
Predictive analytics
Predictive analytics is a powerful tool in medicine, leveraging advanced statistical algorithms to extract valuable insights from patient data and anticipate future outcomes. A variety of ML algorithms, including decision trees, logistic regression, and ensemble methods, are commonly employed. These algorithms analyze historical patient data, incorporating variables such as demographics, medical history, and diagnostic results to predict the likelihood of specific health events. For instance, predictive models might assess the risk of readmission, enabling healthcare providers to implement proactive interventions for high-risk patients. Time-series analysis, often involving algorithms like autoregressive integrated moving averages (ARIMA), is utilized to forecast disease progression and anticipate critical events, supporting timely interventions. Predictive analytics not only aids in risk stratification but also enhances resource allocation, allowing healthcare institutions to optimize workflows and prioritize patient care effectively. As healthcare continues to embrace data-driven approaches, predictive analytics stands as a key driver for personalized and proactive healthcare delivery.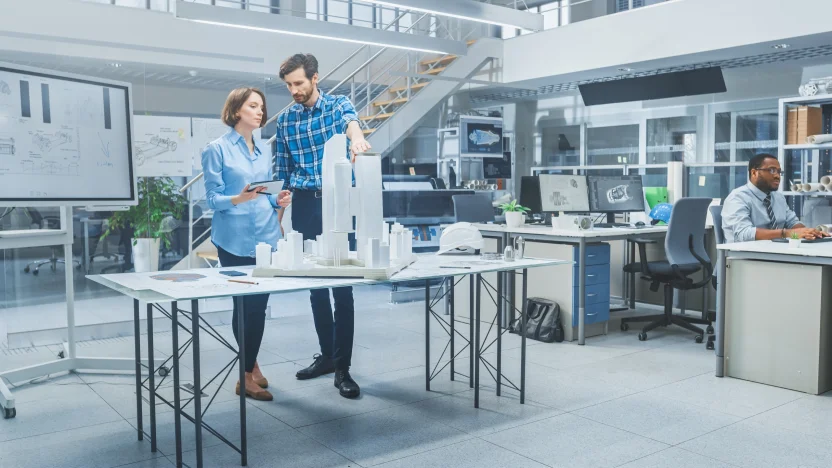
Guidelines for successful implementation of AI in medicine
Define objectives and goals
Clearly outline the objectives of integrating AI into medical practices. Identify specific areas where AI can bring value, such as improving diagnostics, enhancing patient care, or streamlining administrative tasks.
Conduct a needs assessment
Evaluate the current state of healthcare practices and identify areas that could benefit from AI solutions. Engage with healthcare professionals, administrators, and other stakeholders to understand their needs and challenges.
Build a cross-functional team
Assemble a multidisciplinary team that includes healthcare professionals, data scientists, IT specialists, and administrators. This collaborative approach ensures a thorough understanding of both medical requirements and technical considerations.
Develop data governance policies
Establish robust data governance policies to ensure the quality, privacy, and security of patient data. Define data standards, encryption protocols, and access controls to maintain data integrity and compliance with regulations.
Assess data readiness
Evaluate the quality and completeness of existing healthcare data. Ensure that data is appropriately structured, labeled, and available for training AI models. Address any data quality issues before implementing AI solutions.
Select appropriate AI technologies
Choose AI technologies that align with the defined objectives. Consider whether machine learning, natural language processing, robotics, or other AI approaches are most suitable for the identified medical applications.
Build or acquire AI models
Depending on the complexity of the AI solution, organizations can choose to build their own models or acquire pre-trained models. Collaborate with AI developers to customize models based on specific medical requirements.
Ensure interoperability
Prioritize interoperability between AI systems and existing healthcare infrastructure, including Electronic Health Records (EHRs) and clinical systems. Seamless integration enhances efficiency and promotes a cohesive healthcare ecosystem.
Develop and test prototypes
Develop prototypes or minimum viable products (MVPs) to test the feasibility and functionality of the AI solution. Conduct pilot projects in specific healthcare settings to assess performance and gather user feedback.
Address ethical considerations
Develop and enforce ethical guidelines for the use of AI in medicine. Prioritize transparency in algorithmic decision-making, address biases, and ensure patient consent and privacy are central to the AI implementation strategy.
Implement and monitor
Gradually implement AI solutions in real healthcare settings. Monitor the performance of the AI system, gather user feedback, and make iterative improvements. Establish a system for continuous evaluation to adapt to evolving medical needs.
Promote a culture of innovation
Foster a culture of innovation within the organization. Encourage healthcare professionals and AI developers to collaborate on identifying novel applications and solutions that can enhance patient care.
Evaluate and iterate
Regularly evaluate the impact of AI on medical practices. Assess whether the objectives are being met, gather insights on areas of improvement, and iterate on the AI implementation strategy accordingly.
Stay informed on regulatory changes
Stay updated on evolving regulations and standards in the field of AI in medicine. Regularly assess and update AI implementations to ensure compliance with legal and ethical requirements.
By following these steps, healthcare organizations can navigate the complexities of AI integration in medicine and harness the full potential of these technologies to improve healthcare delivery and patient outcomes.
Future trends and possibilities of AI in medicine
Expanded applications in preventive medicine
AI is set to play a pivotal role in preventive medicine by leveraging vast datasets to identify early indicators of disease risk. Predictive analytics will enable healthcare professionals to intervene proactively, potentially averting the onset or progression of various conditions. For instance, AI-powered wearable devices and health monitoring systems will continuously monitor vital signs and detect subtle changes indicative of developing health issues, allowing for timely interventions.
Advanced drug discovery and personalized medicine
The future of drug discovery will witness advancements in AI technologies, facilitating the identification of novel drug candidates and the optimization of treatment plans. AI algorithms will analyze complex biological data, including multi-omics data, to tailor therapies to individual patient characteristics, driving the shift toward personalized medicine. Multi-omics data refers to the integration of various biological datasets, such as genomics, transcriptomics, proteomics, metabolomics, and epigenomics, which provide comprehensive insights into the molecular mechanisms underlying diseases and treatment responses. For example, machine learning models will leverage multi-omics data to predict treatment responses and identify biomarkers for patient stratification, leading to more effective and targeted interventions. By harnessing the power of multi-omics data, AI-driven approaches will revolutionize drug discovery and personalized medicine, paving the way for precision therapies that address the unique biological makeup of each patient.
Enhanced imaging and diagnostics
AI-powered imaging technologies will continue to evolve, offering unprecedented accuracy in disease detection and diagnosis across various medical imaging modalities. Advanced algorithms will enable tasks such as 3D reconstruction, automated lesion segmentation, and real-time image analysis, significantly reducing diagnostic errors and improving treatment planning. For instance, AI-driven radiology systems will provide detailed insights into complex pathologies, aiding clinicians in making more informed decisions and improving patient outcomes.
Explainable AI for clinical decision support
As AI systems become more sophisticated, there will be a growing emphasis on developing explainable AI (XAI) models to enhance transparency and trust in clinical decision-making. Clinicians will have access to intuitive interfaces that provide insights into AI-generated recommendations, enabling them to validate and understand the underlying reasoning. For example, attention mechanisms and interpretable machine learning techniques will empower clinicians to interpret AI-driven diagnoses and treatment suggestions with confidence, fostering a collaborative approach to patient care.
AI in global health initiatives
AI will play a crucial role in addressing global health challenges by enabling more efficient disease surveillance, outbreak prediction, and healthcare delivery in resource-constrained settings. For instance, AI-driven predictive analytics will forecast disease outbreaks and allocate resources effectively, while telemedicine platforms supported by AI will extend healthcare access to underserved populations. However, ensuring equitable access and addressing ethical considerations will be essential for the successful implementation of AI-driven initiatives in diverse cultural and socioeconomic contexts.
Ethical guidelines and regulations
The development of clear ethical guidelines and regulations will be paramount to ensure the responsible and ethical use of AI in healthcare. Regulatory bodies and professional organizations will collaborate to establish frameworks that prioritize patient privacy, transparency, and equity. Interdisciplinary collaborations between ethicists, policymakers, technologists, and healthcare stakeholders will drive the formulation of guidelines that align with ethical principles and patient rights, fostering trust and confidence in AI-driven healthcare solutions. The future of AI in medicine is dynamic and continually evolving, driven by ongoing research, technological advancements, and the collaboration between healthcare professionals and AI experts. As these trends unfold, they are likely to shape a healthcare landscape that is more efficient, personalized, and focused on improving patient outcomes.
Ethical considerations while using AI for medicine
Patient privacy and data security
Patient privacy and data security
Central to the ethical discourse surrounding the utilization of AI in medicine is the protection of patient privacy and the security of healthcare data. As AI algorithms rely on vast amounts of patient data to generate insights and make clinical decisions, ensuring the confidentiality and integrity of this data becomes paramount. Healthcare organizations must implement robust security measures to safeguard sensitive patient information from unauthorized access, breaches, and misuse. Moreover, transparency regarding data collection, storage, and usage practices is essential to foster trust between patients, healthcare providers, and AI systems.
Algorithmic bias and fairness
Algorithmic bias and fairness
Another critical ethical consideration in the utilization of AI in medicine is the presence of algorithmic bias, wherein AI systems may inadvertently perpetuate or amplify existing biases present in the data used for training. Biases related to race, gender, socioeconomic status, and geographic location can lead to disparities in healthcare delivery and outcomes. Addressing algorithmic bias requires careful attention to data selection, algorithm design, and ongoing monitoring to detect and mitigate bias. Additionally, promoting diversity and inclusivity in AI development teams can help mitigate biases and ensure the fairness and equity of AI-driven healthcare solutions.
Healthcare equity and accessibility
Healthcare equity and accessibility
AI has the potential to exacerbate existing disparities in healthcare access and quality if not implemented thoughtfully. While AI-driven technologies hold promise for improving diagnostic accuracy, treatment efficacy, and care coordination, there is a risk of widening the gap between those who have access to advanced AI-driven healthcare solutions and those who do not. To promote healthcare equity and accessibility, stakeholders must prioritize the development and deployment of AI technologies in underserved communities and resource-limited settings. This includes addressing barriers such as digital literacy, language diversity, and cultural sensitivity to ensure that AI-driven healthcare solutions are accessible and inclusive for all individuals, regardless of socioeconomic status or geographic location.
Informed consent and patient autonomy
Informed consent and patient autonomy
In the field of AI-assisted medicine, maintaining patient autonomy and respecting individual preferences are fundamental ethical principles. Patients have the right to understand how AI technologies will impact their care and to make informed decisions about their health based on transparent and understandable information. Healthcare providers must engage patients in meaningful discussions about the use of AI in their treatment, including potential risks, benefits, and alternatives. Informed consent processes should encompass not only the use of AI algorithms in diagnosis and treatment but also the implications for data privacy, security, and confidentiality. Navigating the ethical landscape of medicine enhanced by AI requires a holistic approach that balances innovation with ethical principles, patient-centered care, and societal values. By proactively addressing issues such as patient privacy, algorithmic bias, healthcare equity, and informed consent, stakeholders can harness the transformative potential of AI while upholding ethical standards and promoting the well-being of patients and communities.
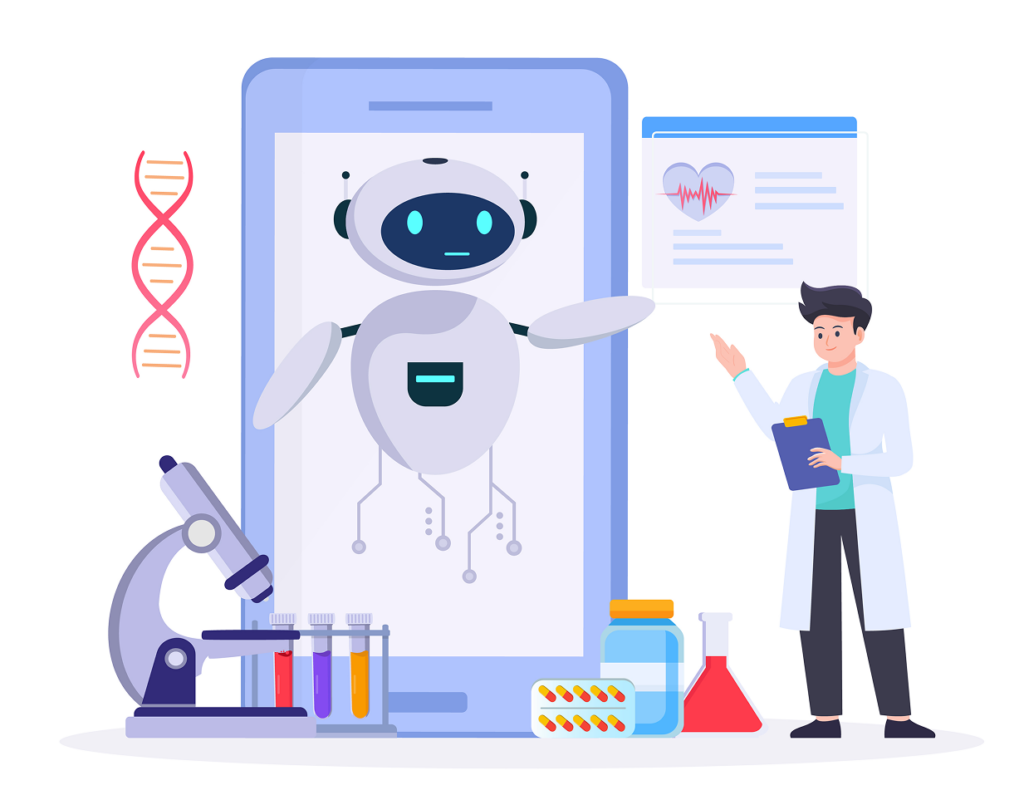
Endnote
The integration of AI and medicine represents an innovative paradigm shift, steering the healthcare industry toward unprecedented heights of efficiency and effectiveness. AI’s transformative influence extends across diverse realms within medicine, from enhancing diagnostic precision to redefining drug discovery and development processes. The landscape of healthcare administration is also undergoing a metamorphosis, with NLP streamlining electronic health records and AI-driven virtual assistants improving patient engagement. As we embrace these technological strides, it is crucial to acknowledge the challenges that accompany such innovations. Ethical considerations, data privacy concerns, and the need for transparent, interpretable algorithms underscore the importance of responsible AI deployment. Striking a balance between technological innovation and ethical considerations is imperative for building a sustainable and patient-centric future for healthcare. Looking forward, the trajectory of AI in medicine holds immense promise. The ongoing collaboration between healthcare professionals, researchers, and AI specialists will be pivotal in unlocking new frontiers. The potential for further integration with robotics, expanded applications in preventive medicine, and continued advancements in drug discovery paints a future where AI is an indispensable ally in the pursuit of better patient outcomes and a more resilient and responsive healthcare ecosystem. In essence, the journey of AI in medicine is a testament to the boundless possibilities that emerge when human ingenuity and technological innovation converge in the noble pursuit of healing and improving lives.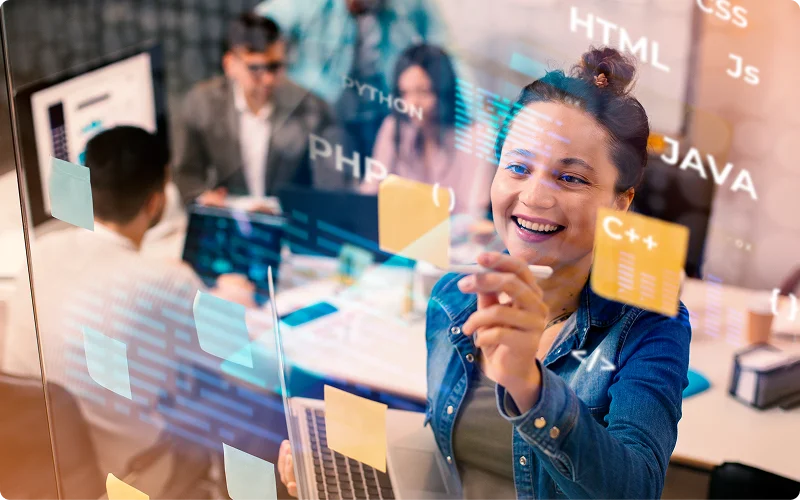
Insights
AI in inventory management: Redefining inventory control for…
AI redefines inventory management, streamlining operations, predicting demand, and optimizing stock control with unrivaled precision and efficiency.
AI in trend analysis: Predicting the pulse of…
In today’s fast-paced, data-driven world, deciphering patterns and trends is more crucial than ever, serving as a compass for organizations and individuals…
A guide to computer vision: Techniques, operational mechanics,…
Computer vision is a field of artificial intelligence that enables computers and systems to derive meaningful insights from digital images, videos, and…